- Volumes 84-95 (2024)
-
Volumes 72-83 (2023)
-
Volume 83
Pages 1-258 (December 2023)
-
Volume 82
Pages 1-204 (November 2023)
-
Volume 81
Pages 1-188 (October 2023)
-
Volume 80
Pages 1-202 (September 2023)
-
Volume 79
Pages 1-172 (August 2023)
-
Volume 78
Pages 1-146 (July 2023)
-
Volume 77
Pages 1-152 (June 2023)
-
Volume 76
Pages 1-176 (May 2023)
-
Volume 75
Pages 1-228 (April 2023)
-
Volume 74
Pages 1-200 (March 2023)
-
Volume 73
Pages 1-138 (February 2023)
-
Volume 72
Pages 1-144 (January 2023)
-
Volume 83
-
Volumes 60-71 (2022)
-
Volume 71
Pages 1-108 (December 2022)
-
Volume 70
Pages 1-106 (November 2022)
-
Volume 69
Pages 1-122 (October 2022)
-
Volume 68
Pages 1-124 (September 2022)
-
Volume 67
Pages 1-102 (August 2022)
-
Volume 66
Pages 1-112 (July 2022)
-
Volume 65
Pages 1-138 (June 2022)
-
Volume 64
Pages 1-186 (May 2022)
-
Volume 63
Pages 1-124 (April 2022)
-
Volume 62
Pages 1-104 (March 2022)
-
Volume 61
Pages 1-120 (February 2022)
-
Volume 60
Pages 1-124 (January 2022)
-
Volume 71
- Volumes 54-59 (2021)
- Volumes 48-53 (2020)
- Volumes 42-47 (2019)
- Volumes 36-41 (2018)
- Volumes 30-35 (2017)
- Volumes 24-29 (2016)
- Volumes 18-23 (2015)
- Volumes 12-17 (2014)
- Volume 11 (2013)
- Volume 10 (2012)
- Volume 9 (2011)
- Volume 8 (2010)
- Volume 7 (2009)
- Volume 6 (2008)
- Volume 5 (2007)
- Volume 4 (2006)
- Volume 3 (2005)
- Volume 2 (2004)
- Volume 1 (2003)
• State of the art review was made for nano-(Q)SAR in silico prediction to toxicity of NMs.
• A critique of measurement methods for physicochemical properties of NMs was provided.
• Computational methods for estimation of physicochemical properties were reviewed.
• Available data in literature was summarized and commented for its usefulness in nano-(Q)SAR.
• Modelling tools that can give source of toxicity and make use of limited data are preferable.
There is increasing recognition that some nanomaterials may pose a risk to human health and the environment. Moreover, the industrial use of the novel engineered nanomaterials (ENMs) increases at a higher rate than data generation for hazard assessment; consequently, many of them remain untested. The large number of nanomaterials and their variants (e.g., different sizes and coatings) requiring testing and the ethical pressure towards nonanimal testing means that in a first instance, expensive animal bioassays are precluded, and the use of (quantitative) structure–activity relationships ((Q)SARs) models as an alternative source of (screening) hazard information should be explored. (Q)SAR modelling can be applied to contribute towards filling important knowledge gaps by making best use of existing data, prioritizing the physicochemical parameters driving toxicity, and providing practical solutions for the risk assessment problems caused by the diversity of ENMs. This paper covers the core components required for successful application of (Q)SAR methods to ENM toxicity prediction, summarizes the published nano-(Q)SAR studies, and outlines the challenges ahead for nano-(Q)SAR modelling. It provides a critical review of (1) the present availability of ENM characterization/toxicity data, (2) the characterization of nanostructures that meet the requirements for (Q)SAR analysis, (3) published nano-(Q)SAR studies and their limitations, (4) in silico tools for (Q)SAR screening of nanotoxicity, and (5) prospective directions for the development of nano-(Q)SAR models.
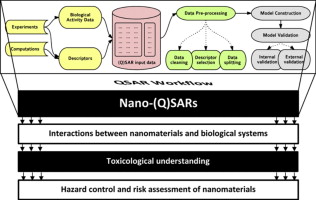