- Volumes 84-95 (2024)
-
Volumes 72-83 (2023)
-
Volume 83
Pages 1-258 (December 2023)
-
Volume 82
Pages 1-204 (November 2023)
-
Volume 81
Pages 1-188 (October 2023)
-
Volume 80
Pages 1-202 (September 2023)
-
Volume 79
Pages 1-172 (August 2023)
-
Volume 78
Pages 1-146 (July 2023)
-
Volume 77
Pages 1-152 (June 2023)
-
Volume 76
Pages 1-176 (May 2023)
-
Volume 75
Pages 1-228 (April 2023)
-
Volume 74
Pages 1-200 (March 2023)
-
Volume 73
Pages 1-138 (February 2023)
-
Volume 72
Pages 1-144 (January 2023)
-
Volume 83
-
Volumes 60-71 (2022)
-
Volume 71
Pages 1-108 (December 2022)
-
Volume 70
Pages 1-106 (November 2022)
-
Volume 69
Pages 1-122 (October 2022)
-
Volume 68
Pages 1-124 (September 2022)
-
Volume 67
Pages 1-102 (August 2022)
-
Volume 66
Pages 1-112 (July 2022)
-
Volume 65
Pages 1-138 (June 2022)
-
Volume 64
Pages 1-186 (May 2022)
-
Volume 63
Pages 1-124 (April 2022)
-
Volume 62
Pages 1-104 (March 2022)
-
Volume 61
Pages 1-120 (February 2022)
-
Volume 60
Pages 1-124 (January 2022)
-
Volume 71
- Volumes 54-59 (2021)
- Volumes 48-53 (2020)
- Volumes 42-47 (2019)
- Volumes 36-41 (2018)
- Volumes 30-35 (2017)
- Volumes 24-29 (2016)
- Volumes 18-23 (2015)
- Volumes 12-17 (2014)
- Volume 11 (2013)
- Volume 10 (2012)
- Volume 9 (2011)
- Volume 8 (2010)
- Volume 7 (2009)
- Volume 6 (2008)
- Volume 5 (2007)
- Volume 4 (2006)
- Volume 3 (2005)
- Volume 2 (2004)
- Volume 1 (2003)
• ANNs and empirical correlations were used to investigate conical spouted beds with draft tubes.
• Both techniques were suitable for predicting average cycle times for data outside the database.
• Dependence of fitting performance on database size was analyzed for the two techniques.
Conventional spouted beds have been extensively used in many real-life applications but are not suited for all types of materials, especially fine particles, which require internal devices to improve their motion in the spouted bed. However, unlike conventional spouted beds, there are almost no mechanistic or empirical models available for the design of spouted beds with internals. Given the availability of an extensive but not experimentally designed database, the main purpose of this study is to present an analysis of neural networks and empirical models in terms of their suitability to fit and predict average cycle times in conical spouted beds with and without draft tubes. The parameters investigated are particle size, density, contactor angle, gas inlet diameter, static bed height, and draft tube features. Although the amount of information is always a key factor when fitting models, the size of the database used in this study strongly affects the fitting performance of empirical models, whereas artificial neural networks are more influenced by how the data are scaled. Results of model verification show that both techniques are suitable for predicting average cycle times for data outside the range covered by the database.
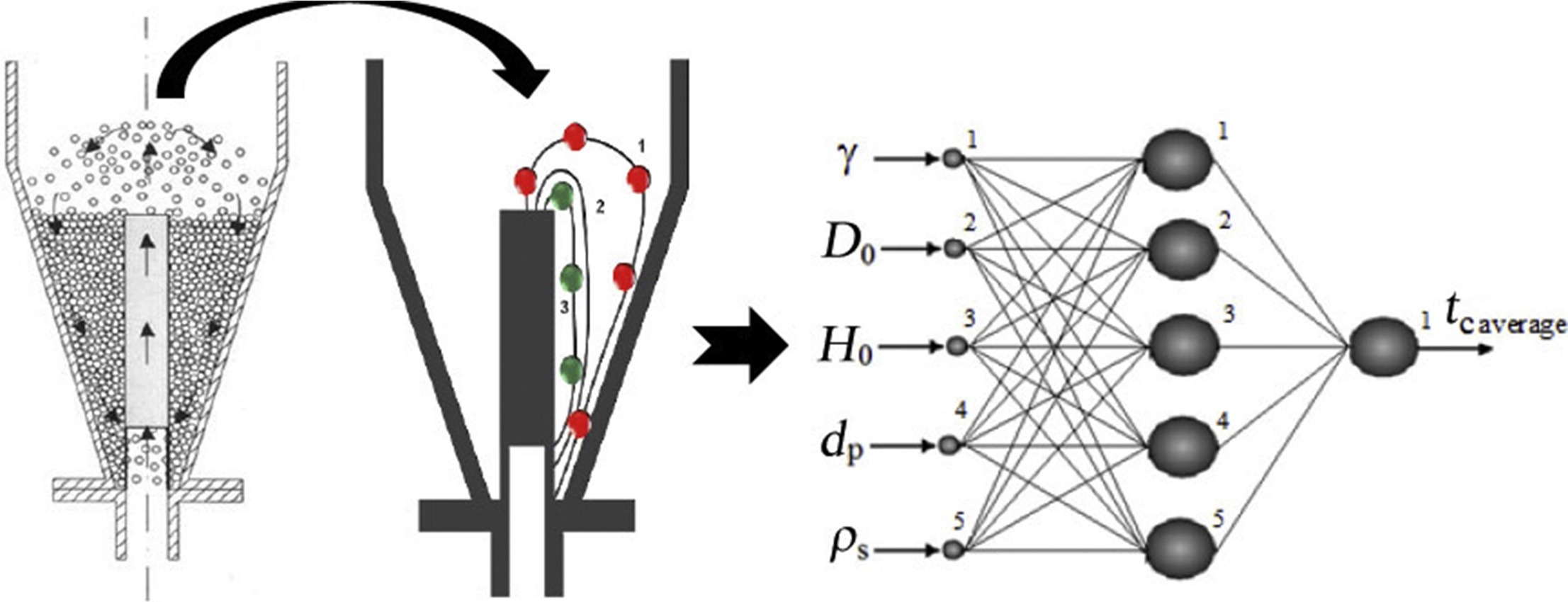