- Volumes 84-95 (2024)
-
Volumes 72-83 (2023)
-
Volume 83
Pages 1-258 (December 2023)
-
Volume 82
Pages 1-204 (November 2023)
-
Volume 81
Pages 1-188 (October 2023)
-
Volume 80
Pages 1-202 (September 2023)
-
Volume 79
Pages 1-172 (August 2023)
-
Volume 78
Pages 1-146 (July 2023)
-
Volume 77
Pages 1-152 (June 2023)
-
Volume 76
Pages 1-176 (May 2023)
-
Volume 75
Pages 1-228 (April 2023)
-
Volume 74
Pages 1-200 (March 2023)
-
Volume 73
Pages 1-138 (February 2023)
-
Volume 72
Pages 1-144 (January 2023)
-
Volume 83
-
Volumes 60-71 (2022)
-
Volume 71
Pages 1-108 (December 2022)
-
Volume 70
Pages 1-106 (November 2022)
-
Volume 69
Pages 1-122 (October 2022)
-
Volume 68
Pages 1-124 (September 2022)
-
Volume 67
Pages 1-102 (August 2022)
-
Volume 66
Pages 1-112 (July 2022)
-
Volume 65
Pages 1-138 (June 2022)
-
Volume 64
Pages 1-186 (May 2022)
-
Volume 63
Pages 1-124 (April 2022)
-
Volume 62
Pages 1-104 (March 2022)
-
Volume 61
Pages 1-120 (February 2022)
-
Volume 60
Pages 1-124 (January 2022)
-
Volume 71
- Volumes 54-59 (2021)
- Volumes 48-53 (2020)
- Volumes 42-47 (2019)
- Volumes 36-41 (2018)
- Volumes 30-35 (2017)
- Volumes 24-29 (2016)
- Volumes 18-23 (2015)
- Volumes 12-17 (2014)
- Volume 11 (2013)
- Volume 10 (2012)
- Volume 9 (2011)
- Volume 8 (2010)
- Volume 7 (2009)
- Volume 6 (2008)
- Volume 5 (2007)
- Volume 4 (2006)
- Volume 3 (2005)
- Volume 2 (2004)
- Volume 1 (2003)
• Signal analyses of acoustic emission (AE) and pressure fluctuation were compared.
• AE signals were analyzed using complexity and Shannon entropy.
• Nonlinear characteristics were studied based on AE signals.
• Flow regime transitions could be identified using AE signal analysis.
Gas–solid flow regimes have a significant impact on particle transport and separation in a fluidized bed reactor. In this study, to determine flow regime transitions in gas–solid fluidized beds, an acoustic technique was used to detect and analyze the behavior of gas and solids. Algorithm complexity, fluctuation complexity, and Shannon entropy analyses of acoustic emission signals were performed to examine nonlinear system characteristics, and to determine the flow regime transition velocities uc, uk, and uFD. Moreover, using the standard deviation of pressure signals, pressure measurements and acoustic measurements were compared. The relative deviations (RDs) between the experimental and empirical values of uk were 8.8%, 13.7%, 8.8%, and 30.4% for the algorithm complexity, fluctuation complexity, Shannon entropy, and pressure signal standard deviation, respectively, while the respective RDs for uFD were 15.7%, 23.9%, 15.7%, and 97.8%. The RDs between the experimental and empirical values of uc were all 6.4%. The experimental values obtained from acoustic signal measurements were therefore closer to the empirical values. In summary, the integration of non-intrusive acoustic measurements, complexity analysis, and Shannon entropy analysis is suitable for identifying flow regime transitions.
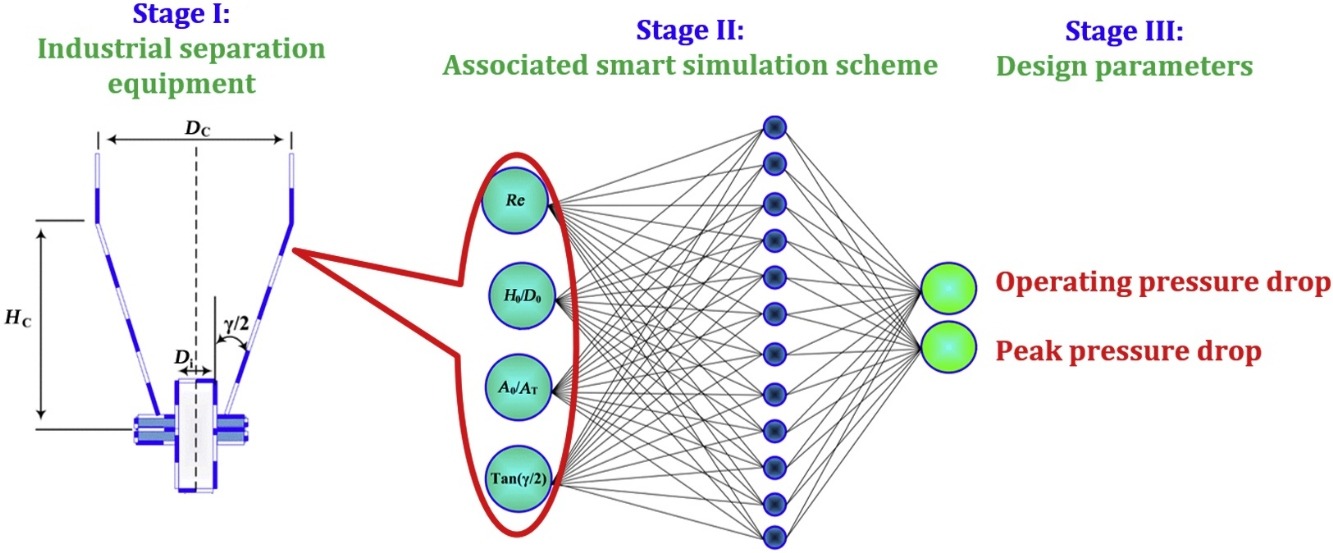