- Volumes 84-95 (2024)
-
Volumes 72-83 (2023)
-
Volume 83
Pages 1-258 (December 2023)
-
Volume 82
Pages 1-204 (November 2023)
-
Volume 81
Pages 1-188 (October 2023)
-
Volume 80
Pages 1-202 (September 2023)
-
Volume 79
Pages 1-172 (August 2023)
-
Volume 78
Pages 1-146 (July 2023)
-
Volume 77
Pages 1-152 (June 2023)
-
Volume 76
Pages 1-176 (May 2023)
-
Volume 75
Pages 1-228 (April 2023)
-
Volume 74
Pages 1-200 (March 2023)
-
Volume 73
Pages 1-138 (February 2023)
-
Volume 72
Pages 1-144 (January 2023)
-
Volume 83
-
Volumes 60-71 (2022)
-
Volume 71
Pages 1-108 (December 2022)
-
Volume 70
Pages 1-106 (November 2022)
-
Volume 69
Pages 1-122 (October 2022)
-
Volume 68
Pages 1-124 (September 2022)
-
Volume 67
Pages 1-102 (August 2022)
-
Volume 66
Pages 1-112 (July 2022)
-
Volume 65
Pages 1-138 (June 2022)
-
Volume 64
Pages 1-186 (May 2022)
-
Volume 63
Pages 1-124 (April 2022)
-
Volume 62
Pages 1-104 (March 2022)
-
Volume 61
Pages 1-120 (February 2022)
-
Volume 60
Pages 1-124 (January 2022)
-
Volume 71
- Volumes 54-59 (2021)
- Volumes 48-53 (2020)
- Volumes 42-47 (2019)
- Volumes 36-41 (2018)
- Volumes 30-35 (2017)
- Volumes 24-29 (2016)
- Volumes 18-23 (2015)
- Volumes 12-17 (2014)
- Volume 11 (2013)
- Volume 10 (2012)
- Volume 9 (2011)
- Volume 8 (2010)
- Volume 7 (2009)
- Volume 6 (2008)
- Volume 5 (2007)
- Volume 4 (2006)
- Volume 3 (2005)
- Volume 2 (2004)
- Volume 1 (2003)
• Conical spouted beds with open-sided draft tubes simulated by a smart model.
• Operating and peak pressure drops accurately estimated by a single approach.
• Overall MSE = 0.00039, AARD% = 1.30, and R2 = 0.7609 obtained for operating pressure drop.
• Overall MSE = 0.22933, AARD% = 11.88, and R2 = 0.8986 obtained for peak pressure drop.
• Guidelines are provided for minimizing both pressure drops in conical spouted beds.
Open-sided draft tubes provide an optimal gas distribution through a cross flow pattern between the spout and the annulus in conical spouted beds. The design, optimization, control, and scale-up of the spouted beds require precise information on operating and peak pressure drops. In this study, a multi-layer perceptron (MLP) neural network was employed for accurate prediction of these hydrodynamic characteristics. A relatively huge number of experiments were accomplished and the most influential dimensionless groups were extracted using the Buckingham-pi theorem. Then, the dimensionless groups were used for developing the MLP model for simultaneous estimation of operating and peak pressure drops. The iterative constructive technique confirmed that 4-14-2 is the best structure for the MLP model in terms of absolute average relative deviation (AARD%), mean square error (MSE), and regression coefficient (R2). The developed MLP approach has an excellent capacity to predict the transformed operating (MSE = 0.00039, AARD% = 1.30, and R2 = 0.76099) and peak (MSE = 0.22933, AARD% = 11.88, and R2 = 0.89867) pressure drops.
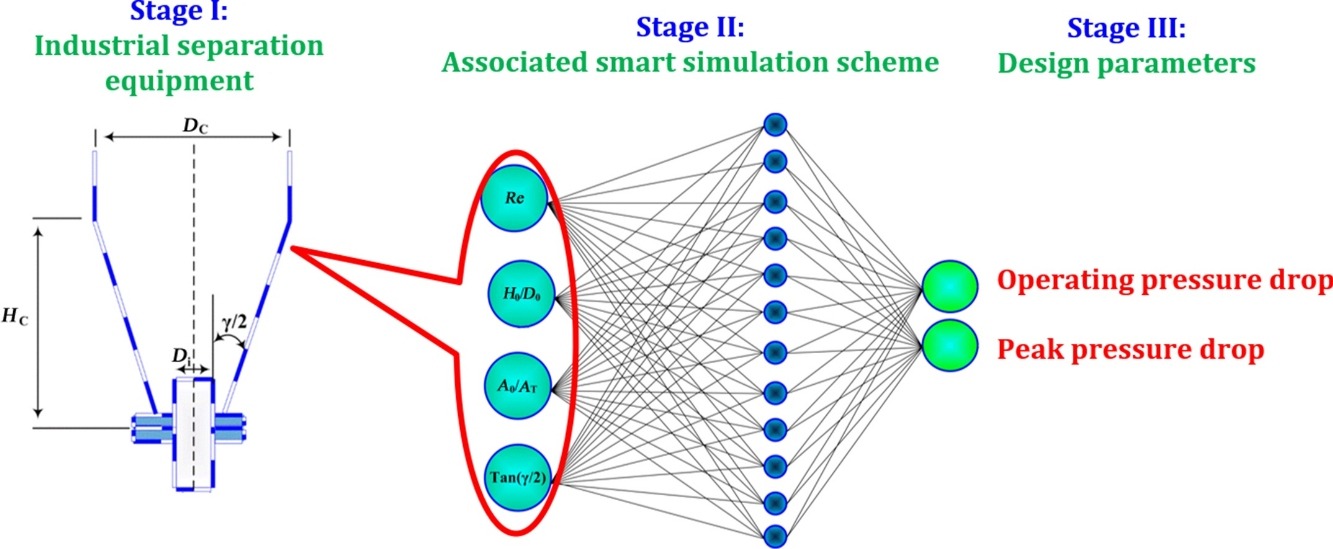