- Volumes 84-95 (2024)
-
Volumes 72-83 (2023)
-
Volume 83
Pages 1-258 (December 2023)
-
Volume 82
Pages 1-204 (November 2023)
-
Volume 81
Pages 1-188 (October 2023)
-
Volume 80
Pages 1-202 (September 2023)
-
Volume 79
Pages 1-172 (August 2023)
-
Volume 78
Pages 1-146 (July 2023)
-
Volume 77
Pages 1-152 (June 2023)
-
Volume 76
Pages 1-176 (May 2023)
-
Volume 75
Pages 1-228 (April 2023)
-
Volume 74
Pages 1-200 (March 2023)
-
Volume 73
Pages 1-138 (February 2023)
-
Volume 72
Pages 1-144 (January 2023)
-
Volume 83
-
Volumes 60-71 (2022)
-
Volume 71
Pages 1-108 (December 2022)
-
Volume 70
Pages 1-106 (November 2022)
-
Volume 69
Pages 1-122 (October 2022)
-
Volume 68
Pages 1-124 (September 2022)
-
Volume 67
Pages 1-102 (August 2022)
-
Volume 66
Pages 1-112 (July 2022)
-
Volume 65
Pages 1-138 (June 2022)
-
Volume 64
Pages 1-186 (May 2022)
-
Volume 63
Pages 1-124 (April 2022)
-
Volume 62
Pages 1-104 (March 2022)
-
Volume 61
Pages 1-120 (February 2022)
-
Volume 60
Pages 1-124 (January 2022)
-
Volume 71
- Volumes 54-59 (2021)
- Volumes 48-53 (2020)
- Volumes 42-47 (2019)
- Volumes 36-41 (2018)
- Volumes 30-35 (2017)
- Volumes 24-29 (2016)
- Volumes 18-23 (2015)
- Volumes 12-17 (2014)
- Volume 11 (2013)
- Volume 10 (2012)
- Volume 9 (2011)
- Volume 8 (2010)
- Volume 7 (2009)
- Volume 6 (2008)
- Volume 5 (2007)
- Volume 4 (2006)
- Volume 3 (2005)
- Volume 2 (2004)
- Volume 1 (2003)
• Particle transport characteristics in branched network were analyzed quantitatively.
• Geometric features of branched networks influenced particle shunting.
• Injection position determined future flow path of particles in the networks.
• Joint influence of inertial, shunt capacity and upstream flow was investigated.
An understanding of the particle transport characteristics in a branched network helps to predict the particle distribution and prevent undesired plugging in various engineering systems. Quantitative analysis of particle flow characteristics is challenging in that experiments are expensive and particle flow is difficult to detect without disturbing the flow. To overcome this difficulty, man-made fractal tree-like branched networks were built, and a coupled computational fluid dynamic and discrete element method model was applied. A series of numerical simulations was carried out to analyze the influence of fractal structure parameters of networks on the particle flow characteristics. The joint influence of inertial, shunt capacity and superposition from upstream branches on particle flow was investigated. The injection position at the inlet determined the particle velocity and its future flow path. The particle density ratio, particle size and bifurcation angle had a greater influence on the shunting of K2 branches than that in the K1 level and Nk22/Nk21 reached a maximum at 60°. Compared with a network with an even number of branches, there was a preferential branch when the branch number was odd. The preferential branch effect or asymmetry degree of the level (K2) branches had a more significant impact on particle shunting than that from the upstream branches (K1).
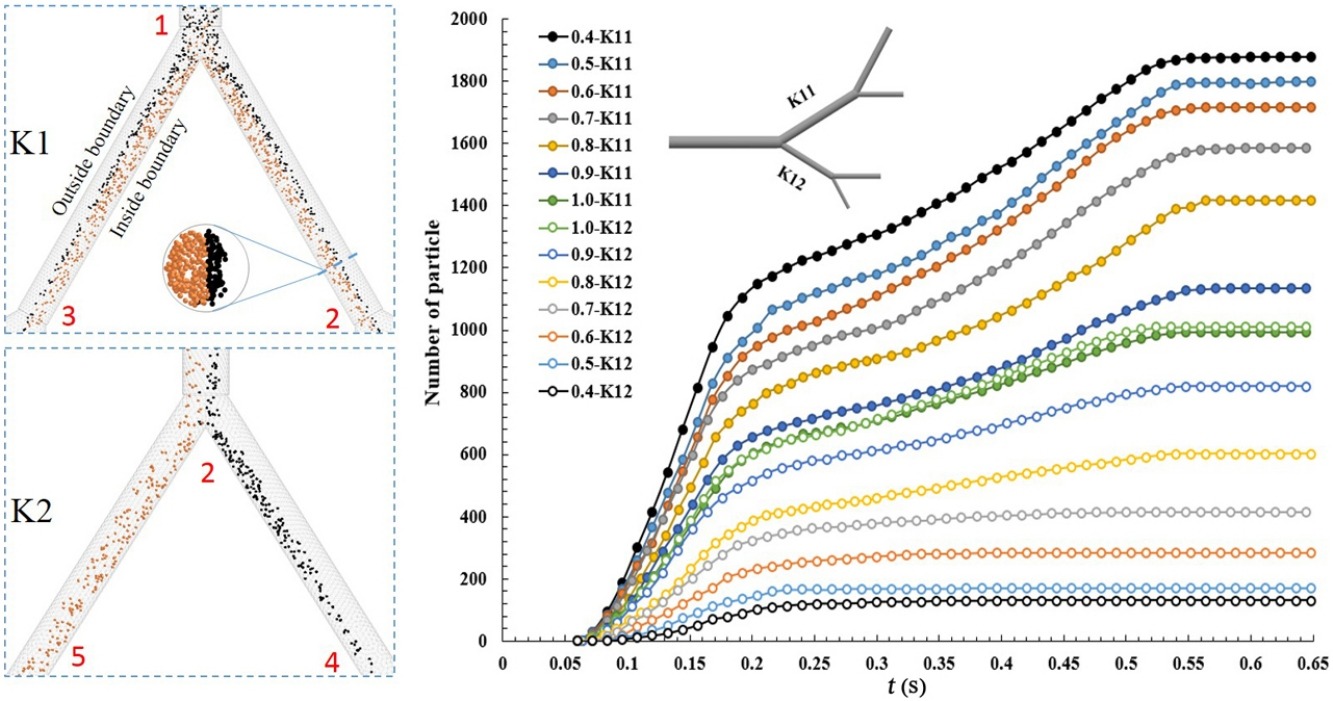