- Volumes 84-95 (2024)
-
Volumes 72-83 (2023)
-
Volume 83
Pages 1-258 (December 2023)
-
Volume 82
Pages 1-204 (November 2023)
-
Volume 81
Pages 1-188 (October 2023)
-
Volume 80
Pages 1-202 (September 2023)
-
Volume 79
Pages 1-172 (August 2023)
-
Volume 78
Pages 1-146 (July 2023)
-
Volume 77
Pages 1-152 (June 2023)
-
Volume 76
Pages 1-176 (May 2023)
-
Volume 75
Pages 1-228 (April 2023)
-
Volume 74
Pages 1-200 (March 2023)
-
Volume 73
Pages 1-138 (February 2023)
-
Volume 72
Pages 1-144 (January 2023)
-
Volume 83
-
Volumes 60-71 (2022)
-
Volume 71
Pages 1-108 (December 2022)
-
Volume 70
Pages 1-106 (November 2022)
-
Volume 69
Pages 1-122 (October 2022)
-
Volume 68
Pages 1-124 (September 2022)
-
Volume 67
Pages 1-102 (August 2022)
-
Volume 66
Pages 1-112 (July 2022)
-
Volume 65
Pages 1-138 (June 2022)
-
Volume 64
Pages 1-186 (May 2022)
-
Volume 63
Pages 1-124 (April 2022)
-
Volume 62
Pages 1-104 (March 2022)
-
Volume 61
Pages 1-120 (February 2022)
-
Volume 60
Pages 1-124 (January 2022)
-
Volume 71
- Volumes 54-59 (2021)
- Volumes 48-53 (2020)
- Volumes 42-47 (2019)
- Volumes 36-41 (2018)
- Volumes 30-35 (2017)
- Volumes 24-29 (2016)
- Volumes 18-23 (2015)
- Volumes 12-17 (2014)
- Volume 11 (2013)
- Volume 10 (2012)
- Volume 9 (2011)
- Volume 8 (2010)
- Volume 7 (2009)
- Volume 6 (2008)
- Volume 5 (2007)
- Volume 4 (2006)
- Volume 3 (2005)
- Volume 2 (2004)
- Volume 1 (2003)
• Pressure drop is an essential parameter in the operation of conical spouted beds.
• Artificial neural networks (ANNs) were used in this study for prediction of operating and peak pressure drops.
• Experimental data fitting of operating and the peak pressure drop was better.
• ANNs have been proven suitable for prediction of pressure drop.
• ANN accuracy is significantly better than empirical correlations.
Pressure drop is an essential parameter in the operation of conical spouted beds (CSB) and depends on its geometric factors and materials used. Irregular materials, like biomass, are complex to treat and, unlike other gas–solid contact methods, CSB turn out to be a suitable technology for their treatment. Artificial neural networks were used in this study for the prediction of operating and peak pressure drops, and their performance has been compared with that of empirical correlations reported in the literature. Accordingly, a multi-layer perceptron network with backward propagation was used due to its ability to model non-linear multivariate systems. The fitting of the experimental data of both operating and peak pressure drop was significantly better than those reported in the literature, specifically in the case of the peak pressure drop, with R2 being 0.92. Therefore, artificial neural networks have been proven suitable for the prediction of pressure drop in CSB.
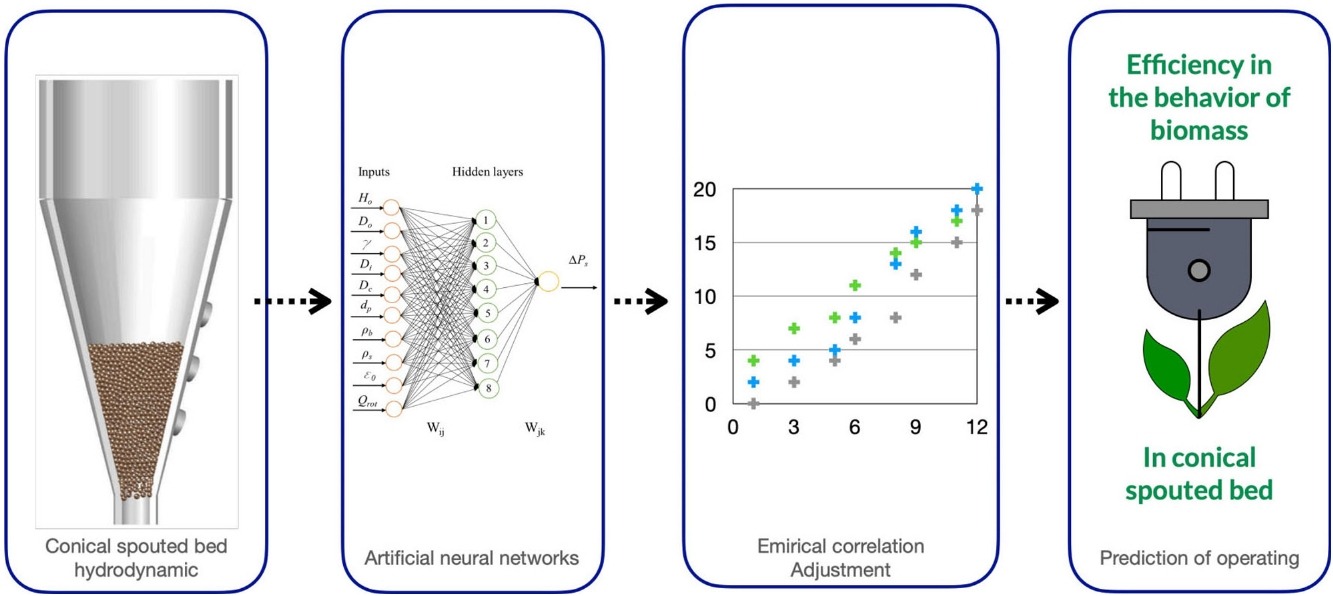