- Volumes 84-95 (2024)
-
Volumes 72-83 (2023)
-
Volume 83
Pages 1-258 (December 2023)
-
Volume 82
Pages 1-204 (November 2023)
-
Volume 81
Pages 1-188 (October 2023)
-
Volume 80
Pages 1-202 (September 2023)
-
Volume 79
Pages 1-172 (August 2023)
-
Volume 78
Pages 1-146 (July 2023)
-
Volume 77
Pages 1-152 (June 2023)
-
Volume 76
Pages 1-176 (May 2023)
-
Volume 75
Pages 1-228 (April 2023)
-
Volume 74
Pages 1-200 (March 2023)
-
Volume 73
Pages 1-138 (February 2023)
-
Volume 72
Pages 1-144 (January 2023)
-
Volume 83
-
Volumes 60-71 (2022)
-
Volume 71
Pages 1-108 (December 2022)
-
Volume 70
Pages 1-106 (November 2022)
-
Volume 69
Pages 1-122 (October 2022)
-
Volume 68
Pages 1-124 (September 2022)
-
Volume 67
Pages 1-102 (August 2022)
-
Volume 66
Pages 1-112 (July 2022)
-
Volume 65
Pages 1-138 (June 2022)
-
Volume 64
Pages 1-186 (May 2022)
-
Volume 63
Pages 1-124 (April 2022)
-
Volume 62
Pages 1-104 (March 2022)
-
Volume 61
Pages 1-120 (February 2022)
-
Volume 60
Pages 1-124 (January 2022)
-
Volume 71
- Volumes 54-59 (2021)
- Volumes 48-53 (2020)
- Volumes 42-47 (2019)
- Volumes 36-41 (2018)
- Volumes 30-35 (2017)
- Volumes 24-29 (2016)
- Volumes 18-23 (2015)
- Volumes 12-17 (2014)
- Volume 11 (2013)
- Volume 10 (2012)
- Volume 9 (2011)
- Volume 8 (2010)
- Volume 7 (2009)
- Volume 6 (2008)
- Volume 5 (2007)
- Volume 4 (2006)
- Volume 3 (2005)
- Volume 2 (2004)
- Volume 1 (2003)
• Deep learning is investigated for crystal segmentation of high solid concentrations.
• Large increase of crystal number with detailed label points enhances model performance.
• Mask R-CNN well recognizes overlapped crystals at high concentrations.
• Mask R-CNN performs much better than multi-scale method in precision and recall.
• Accurate statistics of geometrical features facilitate crystallization understanding.
In situ microscopic imaging is a useful tool in monitoring crystallization processes, including crystal nucleation, growth, aggregation and breakage, as well as possible polymorphic transition. To convert the qualitative information to be quantitative for the purpose of process optimization and control, accurate analysis of crystal images is essential. However, the accuracy of image segmentation with traditional methods is largely affected by many factors, including solid concentration and image quality. In this study, the deep learning technique using mask region-based convolutional neural network (Mask R-CNN) is investigated for the analysis of on-line images from an industrial crystallizer of 10 m3 operated in continuous mode with high solid concentration and overlapped particles. With detailed label points for each crystal and transfer learning technique, two models trained with 70,908 and 7,709 crystals respectively are compared for the effect of training data amount. The former model effectively segments the aggregated and overlapped crystals even at high solid concentrations. Moreover, it performs much better than the latter one and traditional multi-scale method both in terms of precision and recall, revealing the importance of large number of crystals in deep learning. Some geometrical characteristics of segmented crystals are also analyzed, involving equivalent diameter, circularity, and aspect ratio.
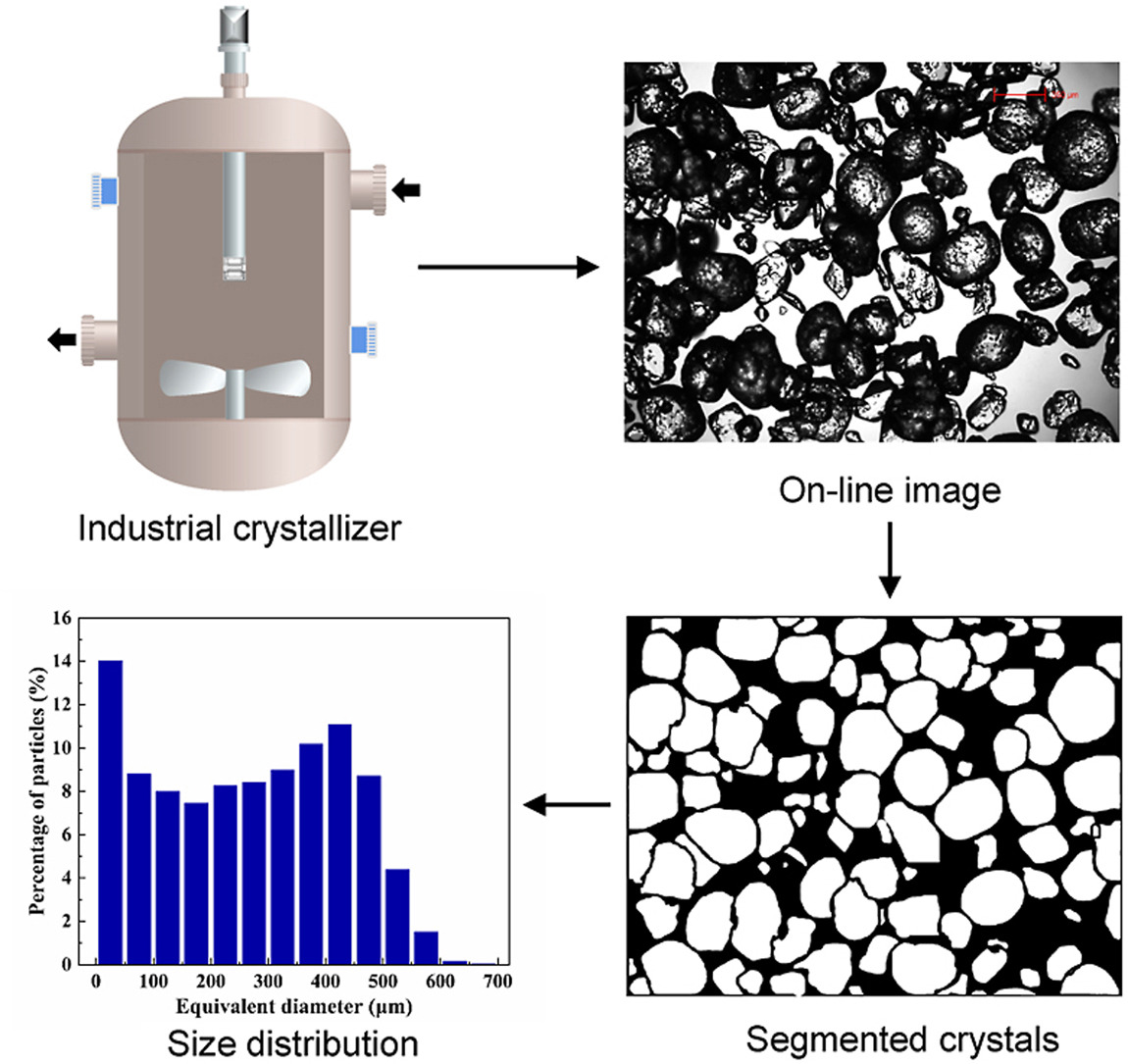