- Volumes 84-95 (2024)
-
Volumes 72-83 (2023)
-
Volume 83
Pages 1-258 (December 2023)
-
Volume 82
Pages 1-204 (November 2023)
-
Volume 81
Pages 1-188 (October 2023)
-
Volume 80
Pages 1-202 (September 2023)
-
Volume 79
Pages 1-172 (August 2023)
-
Volume 78
Pages 1-146 (July 2023)
-
Volume 77
Pages 1-152 (June 2023)
-
Volume 76
Pages 1-176 (May 2023)
-
Volume 75
Pages 1-228 (April 2023)
-
Volume 74
Pages 1-200 (March 2023)
-
Volume 73
Pages 1-138 (February 2023)
-
Volume 72
Pages 1-144 (January 2023)
-
Volume 83
-
Volumes 60-71 (2022)
-
Volume 71
Pages 1-108 (December 2022)
-
Volume 70
Pages 1-106 (November 2022)
-
Volume 69
Pages 1-122 (October 2022)
-
Volume 68
Pages 1-124 (September 2022)
-
Volume 67
Pages 1-102 (August 2022)
-
Volume 66
Pages 1-112 (July 2022)
-
Volume 65
Pages 1-138 (June 2022)
-
Volume 64
Pages 1-186 (May 2022)
-
Volume 63
Pages 1-124 (April 2022)
-
Volume 62
Pages 1-104 (March 2022)
-
Volume 61
Pages 1-120 (February 2022)
-
Volume 60
Pages 1-124 (January 2022)
-
Volume 71
- Volumes 54-59 (2021)
- Volumes 48-53 (2020)
- Volumes 42-47 (2019)
- Volumes 36-41 (2018)
- Volumes 30-35 (2017)
- Volumes 24-29 (2016)
- Volumes 18-23 (2015)
- Volumes 12-17 (2014)
- Volume 11 (2013)
- Volume 10 (2012)
- Volume 9 (2011)
- Volume 8 (2010)
- Volume 7 (2009)
- Volume 6 (2008)
- Volume 5 (2007)
- Volume 4 (2006)
- Volume 3 (2005)
- Volume 2 (2004)
- Volume 1 (2003)
• Investigation of four Machine Learning methods in terms of their ability to model view factors.
• Analysis of markers available in DEM simulations with respect to their view factor correlation.
• DNN- and RFR-based models exceed the quality standards for predictions in monodisperse systems.
• Adding particle size information allows models to change from mono-to polydisperse systems.
We investigated the ability of four popular Machine Learning methods i.e., Deep Neural Networks (DNNs), Random Forest-based regressors (RFRs), Extreme Gradient Boosting-based regressors (XGBs), and stacked ensembles of DNNs, to model the radiative heat transfer based on view factors in bi- and polydisperse particle beds including walls. Before training and analyzing the predictive capability of each method, an adjustment of markers used in monodisperse systems, as well as an evaluation of new markers was performed. On the basis of our dataset that considers a wide range of particle radii ratios, system sizes, particle volume fractions, as well as different particle-species volume fractions, we found that (i) the addition of particle size information allows the transition from monodisperse to bi- and polydisperse beds, and (ii) the addition of particle volume fraction information as the fourth marker leads to very accurate predictions. In terms of the overall performance, DNNs and RFRs should be preferred compared to the other two options. For particle–particle view factors, DNN and RFR are on par, while for particle–wall the RFR is superior. We demonstrate that DNNs and RFRs can be built to meet or even exceed the prediction quality standards achieved in a monodisperse system.
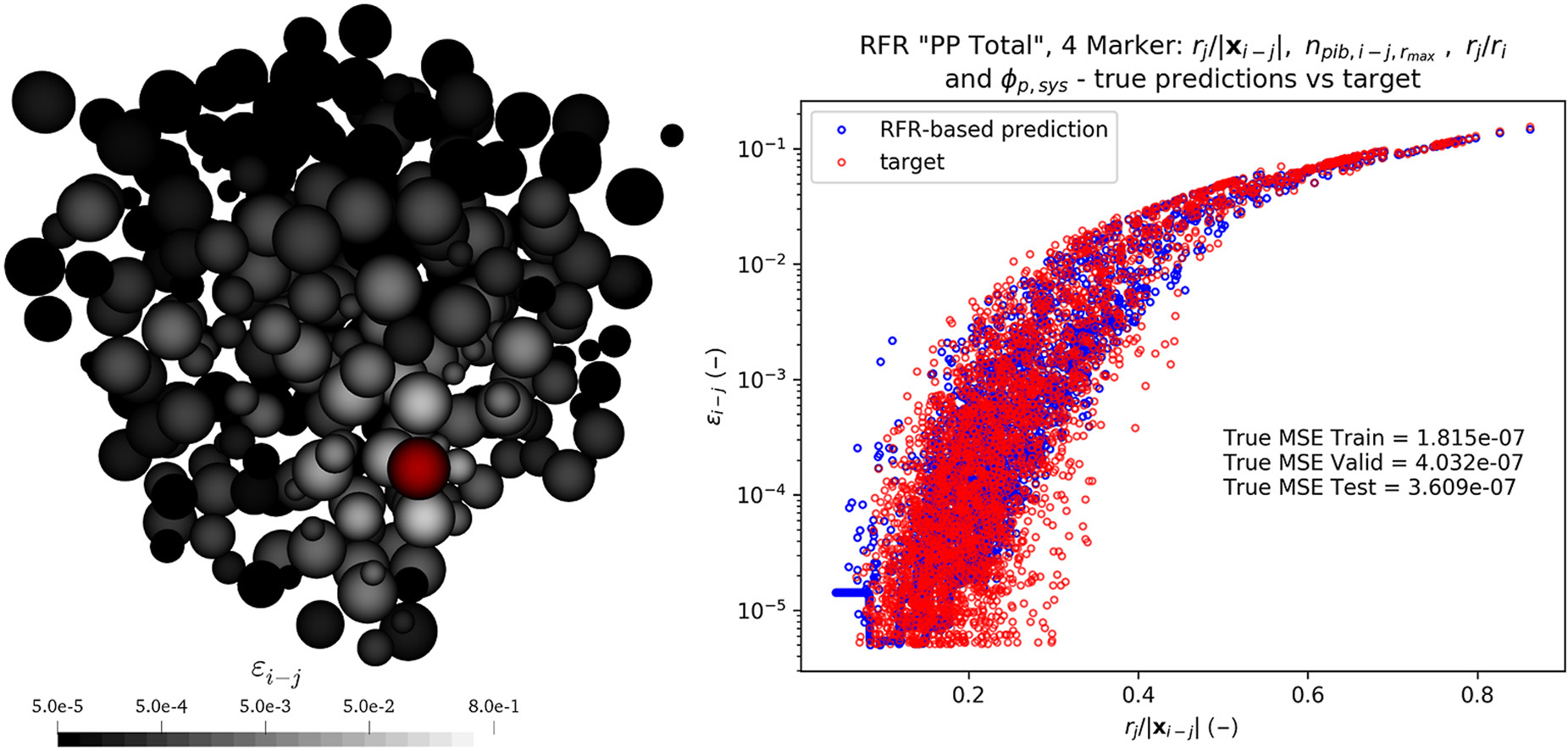