- Volumes 84-95 (2024)
-
Volumes 72-83 (2023)
-
Volume 83
Pages 1-258 (December 2023)
-
Volume 82
Pages 1-204 (November 2023)
-
Volume 81
Pages 1-188 (October 2023)
-
Volume 80
Pages 1-202 (September 2023)
-
Volume 79
Pages 1-172 (August 2023)
-
Volume 78
Pages 1-146 (July 2023)
-
Volume 77
Pages 1-152 (June 2023)
-
Volume 76
Pages 1-176 (May 2023)
-
Volume 75
Pages 1-228 (April 2023)
-
Volume 74
Pages 1-200 (March 2023)
-
Volume 73
Pages 1-138 (February 2023)
-
Volume 72
Pages 1-144 (January 2023)
-
Volume 83
-
Volumes 60-71 (2022)
-
Volume 71
Pages 1-108 (December 2022)
-
Volume 70
Pages 1-106 (November 2022)
-
Volume 69
Pages 1-122 (October 2022)
-
Volume 68
Pages 1-124 (September 2022)
-
Volume 67
Pages 1-102 (August 2022)
-
Volume 66
Pages 1-112 (July 2022)
-
Volume 65
Pages 1-138 (June 2022)
-
Volume 64
Pages 1-186 (May 2022)
-
Volume 63
Pages 1-124 (April 2022)
-
Volume 62
Pages 1-104 (March 2022)
-
Volume 61
Pages 1-120 (February 2022)
-
Volume 60
Pages 1-124 (January 2022)
-
Volume 71
- Volumes 54-59 (2021)
- Volumes 48-53 (2020)
- Volumes 42-47 (2019)
- Volumes 36-41 (2018)
- Volumes 30-35 (2017)
- Volumes 24-29 (2016)
- Volumes 18-23 (2015)
- Volumes 12-17 (2014)
- Volume 11 (2013)
- Volume 10 (2012)
- Volume 9 (2011)
- Volume 8 (2010)
- Volume 7 (2009)
- Volume 6 (2008)
- Volume 5 (2007)
- Volume 4 (2006)
- Volume 3 (2005)
- Volume 2 (2004)
- Volume 1 (2003)
b Department of Mechanical Engineering, National University of Singapore, 117575, Singapore
• An efficient FR-DNS method for particle-laden flows accelerated by multi-GPUs was developed.
• A single GPU V100 achieved 7.1–11.1 times speed up compared to a single CPU (32 cores).
• MPI parallel efficiency achieved 0.68–0.9 for strong scaling on up to 64 DCUs even for dense flow.
• Up to date largest scale gas-solid flow with 1.6 × 109 meshes and 1.6 × 106 particles was simulated on 32 DCUs.
An efficient computing framework, namely PFlows, for fully resolved-direct numerical simulations of particle-laden flows was accelerated on NVIDIA General Processing Units (GPUs) and GPU-like accelerator (DCU) cards. The framework is featured as coupling the lattice Boltzmann method for fluid flow with the immersed boundary method for fluid-particle interaction, and the discrete element method for particle collision, using two fixed Eulerian meshes and one moved Lagrangian point mesh, respectively. All the parts are accelerated by a fine-grained parallelism technique using CUDA on GPUs, and further using HIP on DCU cards, i.e., the calculation on each fluid grid, each immersed boundary point, each particle motion, and each pair-particle collision is responsible by one computer thread, respectively. Coalesced memory accesses to LBM distribution functions with the data layout of Structure of Arrays are used to maximize utilization of hardware bandwidth. Parallel reduction with shared memory for data of immersed boundary points is adopted for the sake of reducing access to global memory when integrate particle hydrodynamic force. MPI computing is further used for computing on heterogeneous architectures with multiple CPUs-GPUs/DCUs. The communications between adjacent processors are hidden by overlapping with calculations. Two benchmark cases were conducted for code validation, including a pure fluid flow and a particle-laden flow. The performances on a single accelerator show that a GPU V100 can achieve 7.1–11.1 times speed up, while a single DCU can achieve 5.6–8.8 times speed up compared to a single Xeon CPU chip (32 cores). The performances on multi-accelerators show that parallel efficiency is 0.5–0.8 for weak scaling and 0.68–0.9 for strong scaling on up to 64 DCU cards even for the dense flow (φ = 20%). The peak performance reaches 179 giga lattice updates per second (GLUPS) on 256 DCU cards by using 1 billion grids and 1 million particles. At last, a large-scale simulation of a gas-solid flow with 1.6 billion grids and 1.6 million particles was conducted using only 32 DCU cards. This simulation shows that the present framework is prospective for simulations of large-scale particle-laden flows in the upcoming exascale computing era.
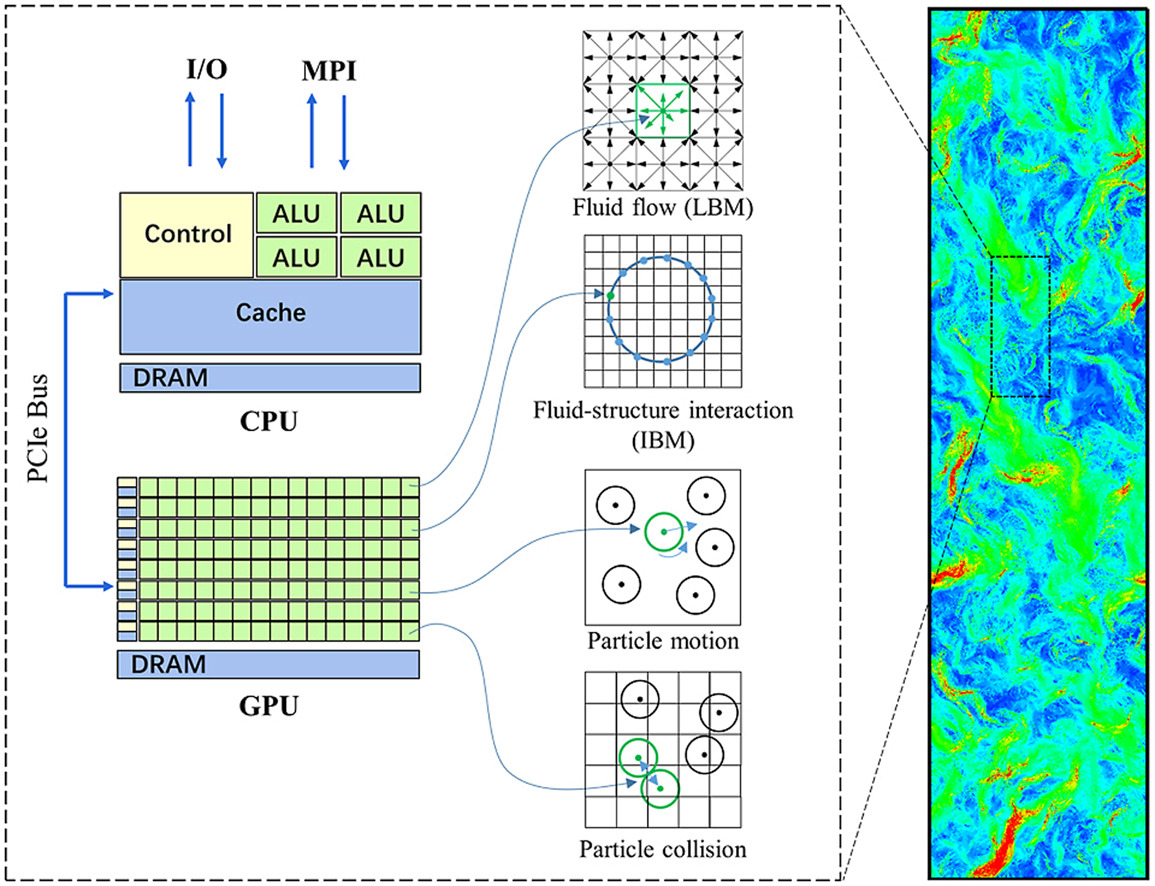