- Volumes 84-95 (2024)
-
Volumes 72-83 (2023)
-
Volume 83
Pages 1-258 (December 2023)
-
Volume 82
Pages 1-204 (November 2023)
-
Volume 81
Pages 1-188 (October 2023)
-
Volume 80
Pages 1-202 (September 2023)
-
Volume 79
Pages 1-172 (August 2023)
-
Volume 78
Pages 1-146 (July 2023)
-
Volume 77
Pages 1-152 (June 2023)
-
Volume 76
Pages 1-176 (May 2023)
-
Volume 75
Pages 1-228 (April 2023)
-
Volume 74
Pages 1-200 (March 2023)
-
Volume 73
Pages 1-138 (February 2023)
-
Volume 72
Pages 1-144 (January 2023)
-
Volume 83
-
Volumes 60-71 (2022)
-
Volume 71
Pages 1-108 (December 2022)
-
Volume 70
Pages 1-106 (November 2022)
-
Volume 69
Pages 1-122 (October 2022)
-
Volume 68
Pages 1-124 (September 2022)
-
Volume 67
Pages 1-102 (August 2022)
-
Volume 66
Pages 1-112 (July 2022)
-
Volume 65
Pages 1-138 (June 2022)
-
Volume 64
Pages 1-186 (May 2022)
-
Volume 63
Pages 1-124 (April 2022)
-
Volume 62
Pages 1-104 (March 2022)
-
Volume 61
Pages 1-120 (February 2022)
-
Volume 60
Pages 1-124 (January 2022)
-
Volume 71
- Volumes 54-59 (2021)
- Volumes 48-53 (2020)
- Volumes 42-47 (2019)
- Volumes 36-41 (2018)
- Volumes 30-35 (2017)
- Volumes 24-29 (2016)
- Volumes 18-23 (2015)
- Volumes 12-17 (2014)
- Volume 11 (2013)
- Volume 10 (2012)
- Volume 9 (2011)
- Volume 8 (2010)
- Volume 7 (2009)
- Volume 6 (2008)
- Volume 5 (2007)
- Volume 4 (2006)
- Volume 3 (2005)
- Volume 2 (2004)
- Volume 1 (2003)
• Neural network-based parameter identification of diffusion coefficients from high-resolution pore network model simulations.
• Model reduction of pore network models and non-intrusive simulation of the time evolution using neural networks.
• Speed-up of multiple orders of magnitude for both approaches.
Reacting particle systems play an important role in many industrial applications, for example biomass drying or the manufacturing of pharmaceuticals. The numerical modeling and simulation of such systems is therefore of great importance for an efficient, reliable, and environmentally sustainable operation of the processes. The complex thermodynamical, chemical, and flow processes that take place in the particles are a particular challenge in a simulation. Furthermore, typically a large number of particles is involved, rendering an explicit treatment of individual ones impossible in a reactor-level simulation. One approach for overcoming this challenge is to compute effective, physical parameters from single-particle, high-resolution simulations. This can be combined with model reduction methods if the dynamical behaviour of particles must be captured. Pore network models with their unrivaled resolution have thereby been used successfully as high-resolution models, for instance to obtain the macroscopic diffusion coefficient of drying. Both parameter identification and model reduction have recently gained new impetus by the dramatic progress made in machine learning in the last decade. We report results on the use of neural networks for parameter identification and model reduction based on three-dimensional pore network models (PNM). We believe that our results provide a powerful complement to existing methodologies for reactor-level simulations with many thermally-thick particles.
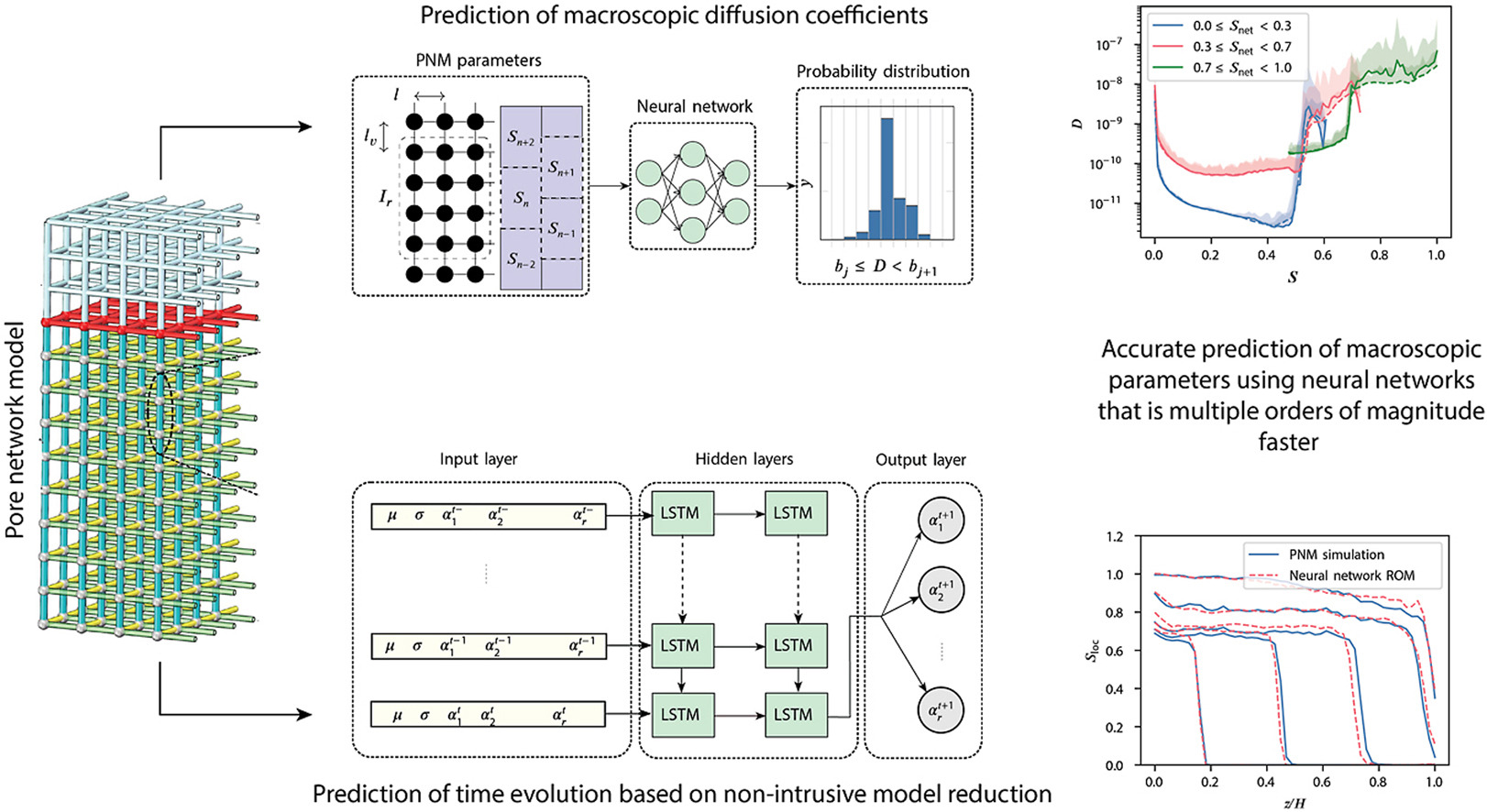