- Volumes 84-95 (2024)
-
Volumes 72-83 (2023)
-
Volume 83
Pages 1-258 (December 2023)
-
Volume 82
Pages 1-204 (November 2023)
-
Volume 81
Pages 1-188 (October 2023)
-
Volume 80
Pages 1-202 (September 2023)
-
Volume 79
Pages 1-172 (August 2023)
-
Volume 78
Pages 1-146 (July 2023)
-
Volume 77
Pages 1-152 (June 2023)
-
Volume 76
Pages 1-176 (May 2023)
-
Volume 75
Pages 1-228 (April 2023)
-
Volume 74
Pages 1-200 (March 2023)
-
Volume 73
Pages 1-138 (February 2023)
-
Volume 72
Pages 1-144 (January 2023)
-
Volume 83
-
Volumes 60-71 (2022)
-
Volume 71
Pages 1-108 (December 2022)
-
Volume 70
Pages 1-106 (November 2022)
-
Volume 69
Pages 1-122 (October 2022)
-
Volume 68
Pages 1-124 (September 2022)
-
Volume 67
Pages 1-102 (August 2022)
-
Volume 66
Pages 1-112 (July 2022)
-
Volume 65
Pages 1-138 (June 2022)
-
Volume 64
Pages 1-186 (May 2022)
-
Volume 63
Pages 1-124 (April 2022)
-
Volume 62
Pages 1-104 (March 2022)
-
Volume 61
Pages 1-120 (February 2022)
-
Volume 60
Pages 1-124 (January 2022)
-
Volume 71
- Volumes 54-59 (2021)
- Volumes 48-53 (2020)
- Volumes 42-47 (2019)
- Volumes 36-41 (2018)
- Volumes 30-35 (2017)
- Volumes 24-29 (2016)
- Volumes 18-23 (2015)
- Volumes 12-17 (2014)
- Volume 11 (2013)
- Volume 10 (2012)
- Volume 9 (2011)
- Volume 8 (2010)
- Volume 7 (2009)
- Volume 6 (2008)
- Volume 5 (2007)
- Volume 4 (2006)
- Volume 3 (2005)
- Volume 2 (2004)
- Volume 1 (2003)
• Kinetic model of vibration screening using bio-inspired neural network is proposed.
• Differential equation describing the neural dynamic characteristics is derived.
• Relationship between actual screening parameters and model coefficients is established.
• Feasibility and advantage of the proposed method are demonstrated.
The kinetic model is the theoretical basis for optimizing the structure and operation performance of vibration screening devices. In this paper, a biological neurodynamic equation and neural connections were established according to the motion and interaction properties of the material under vibration excitation. The material feeding to the screen and the material passing through apertures were considered as excitatory and inhibitory inputs, respectively, and the generated stable neural activity landscape was used to describe the material distribution on the 2D screen surface. The dynamic process of material vibration screening was simulated using discrete element method (DEM). By comparing the similarity between the material distribution established using biological neural network (BNN) and that obtained using DEM simulation, the optimum coefficients of BNN model under a certain screening parameter were determined, that is, one relationship between the BNN model coefficients and the screening operation parameters was established. Different screening parameters were randomly selected, and the corresponding relationships were established as a database. Then, with straw/grain ratio, aperture diameter, inclination angle, vibration strength in normal and tangential directions as inputs, five independent adaptive neuro-fuzzy inference systems (ANFIS) were established to predict the optimum BNN model coefficients, respectively. The training results indicated that ANFIS models had good stability and accuracy. The flexibility and adaptability of the proposed BNN method was demonstrated by modeling material distribution under complex feeding conditions such as multiple regions and non-uniform rate.
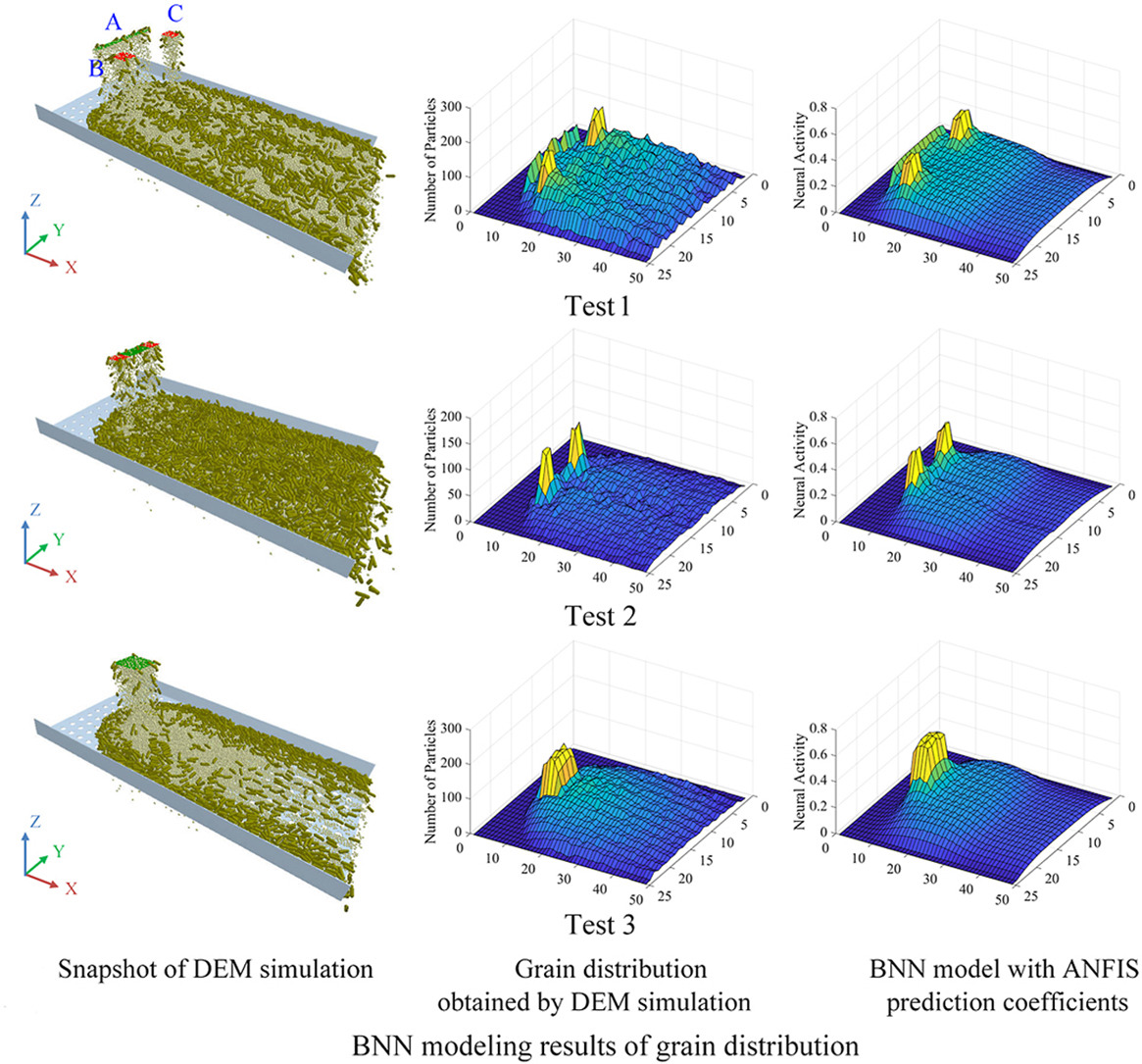