- Volumes 84-95 (2024)
-
Volumes 72-83 (2023)
-
Volume 83
Pages 1-258 (December 2023)
-
Volume 82
Pages 1-204 (November 2023)
-
Volume 81
Pages 1-188 (October 2023)
-
Volume 80
Pages 1-202 (September 2023)
-
Volume 79
Pages 1-172 (August 2023)
-
Volume 78
Pages 1-146 (July 2023)
-
Volume 77
Pages 1-152 (June 2023)
-
Volume 76
Pages 1-176 (May 2023)
-
Volume 75
Pages 1-228 (April 2023)
-
Volume 74
Pages 1-200 (March 2023)
-
Volume 73
Pages 1-138 (February 2023)
-
Volume 72
Pages 1-144 (January 2023)
-
Volume 83
-
Volumes 60-71 (2022)
-
Volume 71
Pages 1-108 (December 2022)
-
Volume 70
Pages 1-106 (November 2022)
-
Volume 69
Pages 1-122 (October 2022)
-
Volume 68
Pages 1-124 (September 2022)
-
Volume 67
Pages 1-102 (August 2022)
-
Volume 66
Pages 1-112 (July 2022)
-
Volume 65
Pages 1-138 (June 2022)
-
Volume 64
Pages 1-186 (May 2022)
-
Volume 63
Pages 1-124 (April 2022)
-
Volume 62
Pages 1-104 (March 2022)
-
Volume 61
Pages 1-120 (February 2022)
-
Volume 60
Pages 1-124 (January 2022)
-
Volume 71
- Volumes 54-59 (2021)
- Volumes 48-53 (2020)
- Volumes 42-47 (2019)
- Volumes 36-41 (2018)
- Volumes 30-35 (2017)
- Volumes 24-29 (2016)
- Volumes 18-23 (2015)
- Volumes 12-17 (2014)
- Volume 11 (2013)
- Volume 10 (2012)
- Volume 9 (2011)
- Volume 8 (2010)
- Volume 7 (2009)
- Volume 6 (2008)
- Volume 5 (2007)
- Volume 4 (2006)
- Volume 3 (2005)
- Volume 2 (2004)
- Volume 1 (2003)
• Industrial reactor optimization is realized with simulation and machine learning.
• Performance data set is constructed with simulations of 1500 cases of an methanol to olefins reactor.
• Ensemble learning and k-fold cross validation is used to enhance prediction accuracy.
• Particle swarm optimization is coupled to search operations for specific performance.
Design, scaling-up, and optimization of industrial reactors mainly depend on step-by-step experiments and engineering experience, which is usually time-consuming, high cost, and high risk. Although numerical simulation can reproduce high resolution details of hydrodynamics, thermal transfer, and reaction process in reactors, it is still challenging for industrial reactors due to huge computational cost. In this study, by combining the numerical simulation and artificial intelligence (AI) technology of machine learning (ML), a method is proposed to efficiently predict and optimize the performance of industrial reactors. A gas–solid fluidization reactor for the methanol to olefins process is taken as an example. 1500 cases under different conditions are simulated by the coarse-grain discrete particle method based on the Energy-Minimization Multi-Scale model, and thus, the reactor performance data set is constructed. To develop an efficient reactor performance prediction model influenced by multiple factors, the ML method is established including the ensemble learning strategy and automatic hyperparameter optimization technique, which has better performance than the methods based on the artificial neural network. Furthermore, the operating conditions for highest yield of ethylene and propylene or lowest pressure drop are searched with the particle swarm optimization algorithm due to its strength to solve non-linear optimization problems. Results show that decreasing the methanol inflow rate and increasing the catalyst inventory can maximize the yield, while decreasing methanol the inflow rate and reducing the catalyst inventory can minimize the pressure drop. The two objectives are thus conflicting, and the practical operations need to be compromised under different circumstance.
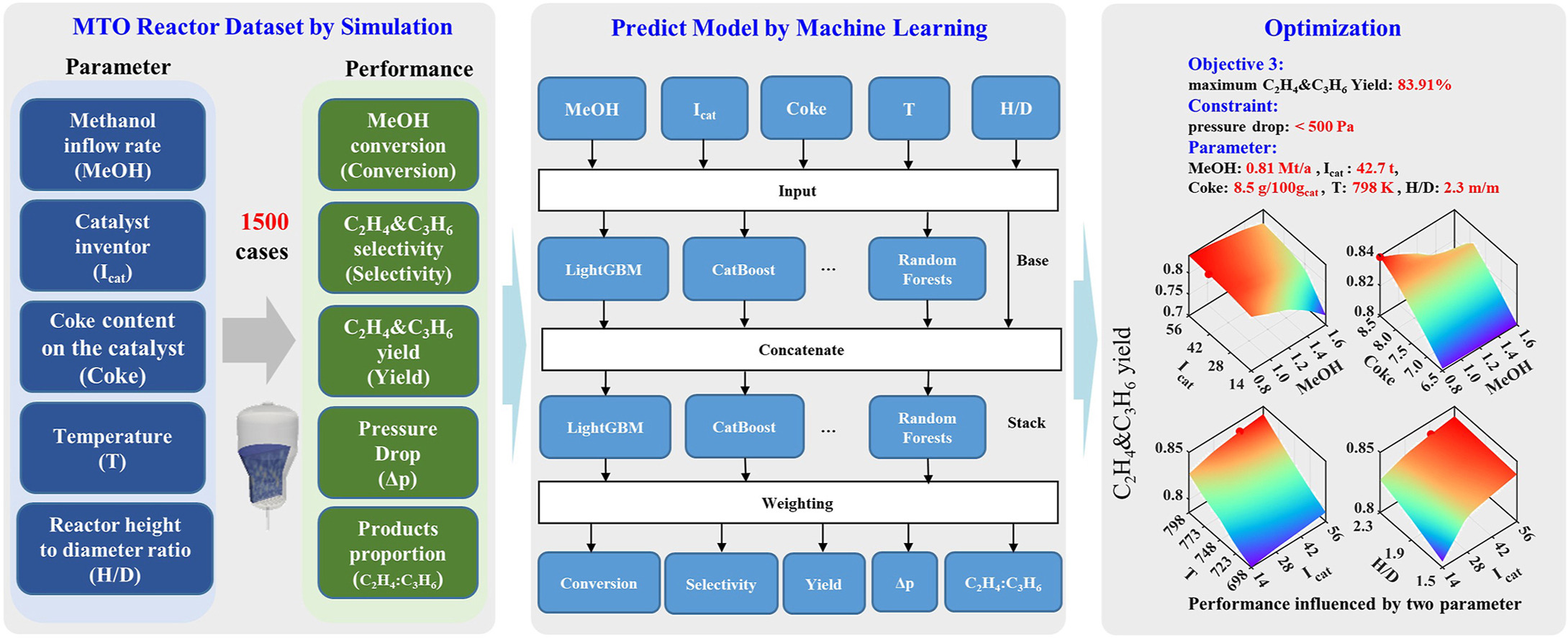