- Volumes 84-95 (2024)
-
Volumes 72-83 (2023)
-
Volume 83
Pages 1-258 (December 2023)
-
Volume 82
Pages 1-204 (November 2023)
-
Volume 81
Pages 1-188 (October 2023)
-
Volume 80
Pages 1-202 (September 2023)
-
Volume 79
Pages 1-172 (August 2023)
-
Volume 78
Pages 1-146 (July 2023)
-
Volume 77
Pages 1-152 (June 2023)
-
Volume 76
Pages 1-176 (May 2023)
-
Volume 75
Pages 1-228 (April 2023)
-
Volume 74
Pages 1-200 (March 2023)
-
Volume 73
Pages 1-138 (February 2023)
-
Volume 72
Pages 1-144 (January 2023)
-
Volume 83
-
Volumes 60-71 (2022)
-
Volume 71
Pages 1-108 (December 2022)
-
Volume 70
Pages 1-106 (November 2022)
-
Volume 69
Pages 1-122 (October 2022)
-
Volume 68
Pages 1-124 (September 2022)
-
Volume 67
Pages 1-102 (August 2022)
-
Volume 66
Pages 1-112 (July 2022)
-
Volume 65
Pages 1-138 (June 2022)
-
Volume 64
Pages 1-186 (May 2022)
-
Volume 63
Pages 1-124 (April 2022)
-
Volume 62
Pages 1-104 (March 2022)
-
Volume 61
Pages 1-120 (February 2022)
-
Volume 60
Pages 1-124 (January 2022)
-
Volume 71
- Volumes 54-59 (2021)
- Volumes 48-53 (2020)
- Volumes 42-47 (2019)
- Volumes 36-41 (2018)
- Volumes 30-35 (2017)
- Volumes 24-29 (2016)
- Volumes 18-23 (2015)
- Volumes 12-17 (2014)
- Volume 11 (2013)
- Volume 10 (2012)
- Volume 9 (2011)
- Volume 8 (2010)
- Volume 7 (2009)
- Volume 6 (2008)
- Volume 5 (2007)
- Volume 4 (2006)
- Volume 3 (2005)
- Volume 2 (2004)
- Volume 1 (2003)
• Examined the blending of two types of cohesive powder in a mixture.
• Validated discrete element method (DEM) model for a lab-scale single-paddle blender with 3.47% relative error.
• Investigated the impact of various operating parameters on mixing quality.
• Highlighted the promise of employing machine learning in analyzing DEM outcomes.
• Introduced an machine learning model for the mixing index with an mean absolute error value of 0.0183.
This research paper presents a comprehensive discrete element method (DEM) examination of the mixing behaviors exhibited by cohesive particles within a twin-paddle blender. A comparative analysis between the simulation and experimental results revealed a relative error of 3.47%, demonstrating a strong agreement between the results from the experimental tests and the DEM simulation. The main focus centers on systematically exploring how operational parameters, such as impeller rotational speed, blender's fill level, and particle mass ratio, influence the process. The investigation also illustrates the significant influence of the mixing time on the mixing quality. To gain a deeper understanding of the DEM simulation findings, an analytical tool called multivariate polynomial regression in machine learning is employed. This method uncovers significant connections between the DEM results and the operational parameters, providing a more comprehensive insight into their interrelationships. The multivariate polynomial regression model exhibited robust predictive performance, with a mean absolute percentage error of less than 3% for both the training and validation sets, indicating a slight deviation from actual values. The model's precision was confirmed by low mean absolute error values of 0.0144 (80% of the dataset in the training set) and 0.0183 (20% of the dataset in the validation set). The study offers valuable insights into granular mixing behaviors, with implications for enhancing the efficiency and predictability of the mixing processes in various industrial applications.
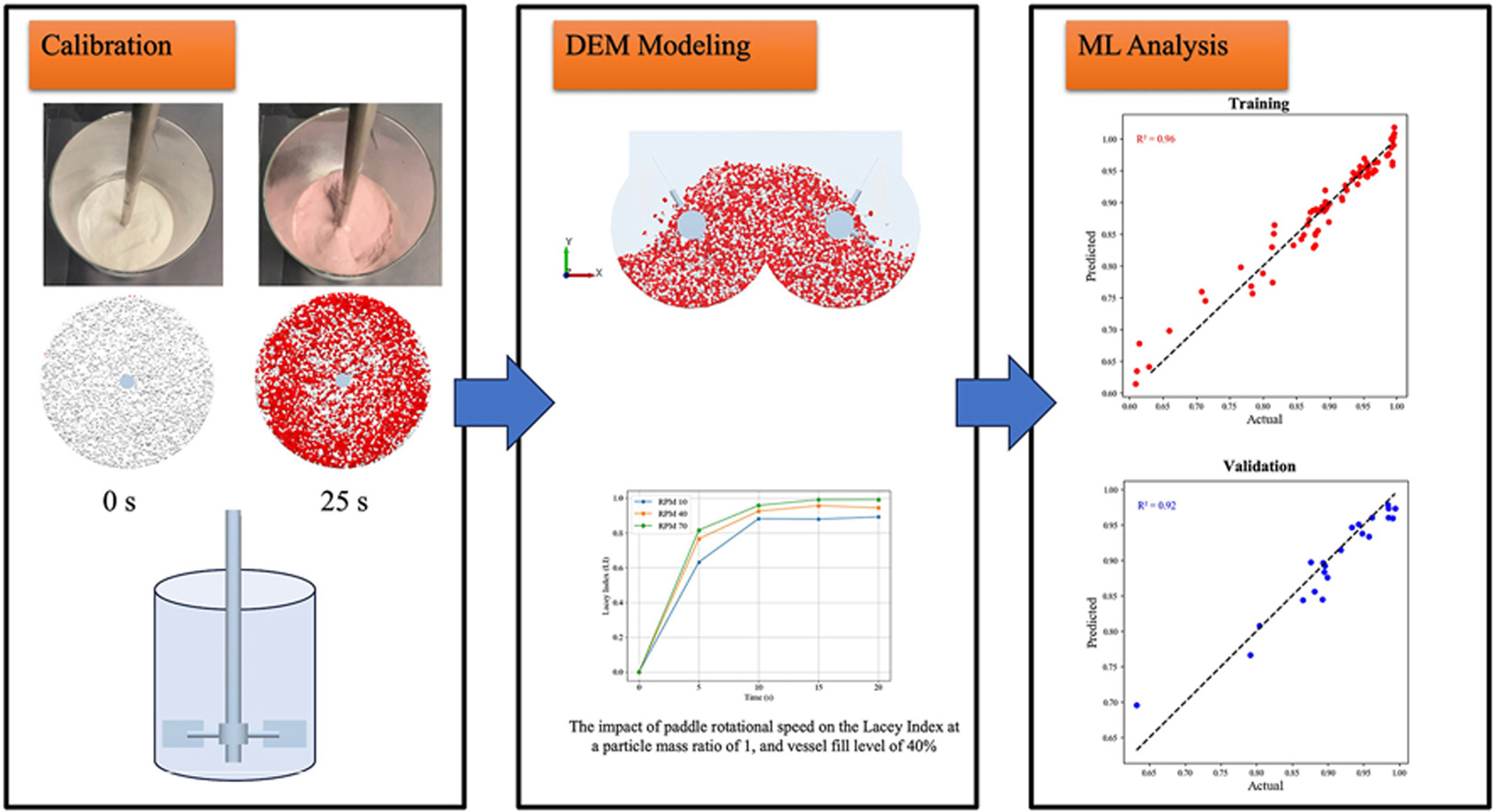