- Volumes 84-95 (2024)
-
Volumes 72-83 (2023)
-
Volume 83
Pages 1-258 (December 2023)
-
Volume 82
Pages 1-204 (November 2023)
-
Volume 81
Pages 1-188 (October 2023)
-
Volume 80
Pages 1-202 (September 2023)
-
Volume 79
Pages 1-172 (August 2023)
-
Volume 78
Pages 1-146 (July 2023)
-
Volume 77
Pages 1-152 (June 2023)
-
Volume 76
Pages 1-176 (May 2023)
-
Volume 75
Pages 1-228 (April 2023)
-
Volume 74
Pages 1-200 (March 2023)
-
Volume 73
Pages 1-138 (February 2023)
-
Volume 72
Pages 1-144 (January 2023)
-
Volume 83
-
Volumes 60-71 (2022)
-
Volume 71
Pages 1-108 (December 2022)
-
Volume 70
Pages 1-106 (November 2022)
-
Volume 69
Pages 1-122 (October 2022)
-
Volume 68
Pages 1-124 (September 2022)
-
Volume 67
Pages 1-102 (August 2022)
-
Volume 66
Pages 1-112 (July 2022)
-
Volume 65
Pages 1-138 (June 2022)
-
Volume 64
Pages 1-186 (May 2022)
-
Volume 63
Pages 1-124 (April 2022)
-
Volume 62
Pages 1-104 (March 2022)
-
Volume 61
Pages 1-120 (February 2022)
-
Volume 60
Pages 1-124 (January 2022)
-
Volume 71
- Volumes 54-59 (2021)
- Volumes 48-53 (2020)
- Volumes 42-47 (2019)
- Volumes 36-41 (2018)
- Volumes 30-35 (2017)
- Volumes 24-29 (2016)
- Volumes 18-23 (2015)
- Volumes 12-17 (2014)
- Volume 11 (2013)
- Volume 10 (2012)
- Volume 9 (2011)
- Volume 8 (2010)
- Volume 7 (2009)
- Volume 6 (2008)
- Volume 5 (2007)
- Volume 4 (2006)
- Volume 3 (2005)
- Volume 2 (2004)
- Volume 1 (2003)
• A novel nonintrusive reduced-order modeling method is proposed.
• Dynamic evolution characteristics of fluidized bed flow fields are captured.
• Computational efficiency is improved by five orders of magnitude.
The fast and accurate reduced-order modeling of fluidized beds is a challenging task in the field of fluid dynamics, owing to their high dimensionality and nonlinear dynamic behavior. In this study, a nonintrusive reduced order modeling method, the reduced order model based on principal component analysis and bidirectional long short-term memory networks (PBLSTM ROM), was developed to capture complex spatio-temporal dynamics of fluidized beds. By combining principal component analysis and Bidirectional long- short-term memory networks, the PBLSTM ROM effectively extracted dynamic evolution information without any prior knowledge of governing equations, enabling reduced-order modeling of unsteady flow fields. The PBLSTM ROM was validated using the solid volume fraction and gas velocity flow fields of a fluidized bed with immersed tubes, showing superior performance over both the PLSTM and PANN ROMs in accurately capturing temporal changes in the fluidization fields, especially in the region near immersed tubes where severe fluctuations appear. Moreover, the PBLSTM ROM improved the simulation speed by five orders of magnitude compared to traditional computational fluid dynamics simulations. These findings suggest that the PBLSTM ROM presents a promising approach for analyzing the complex fluid flows in engineering practice.
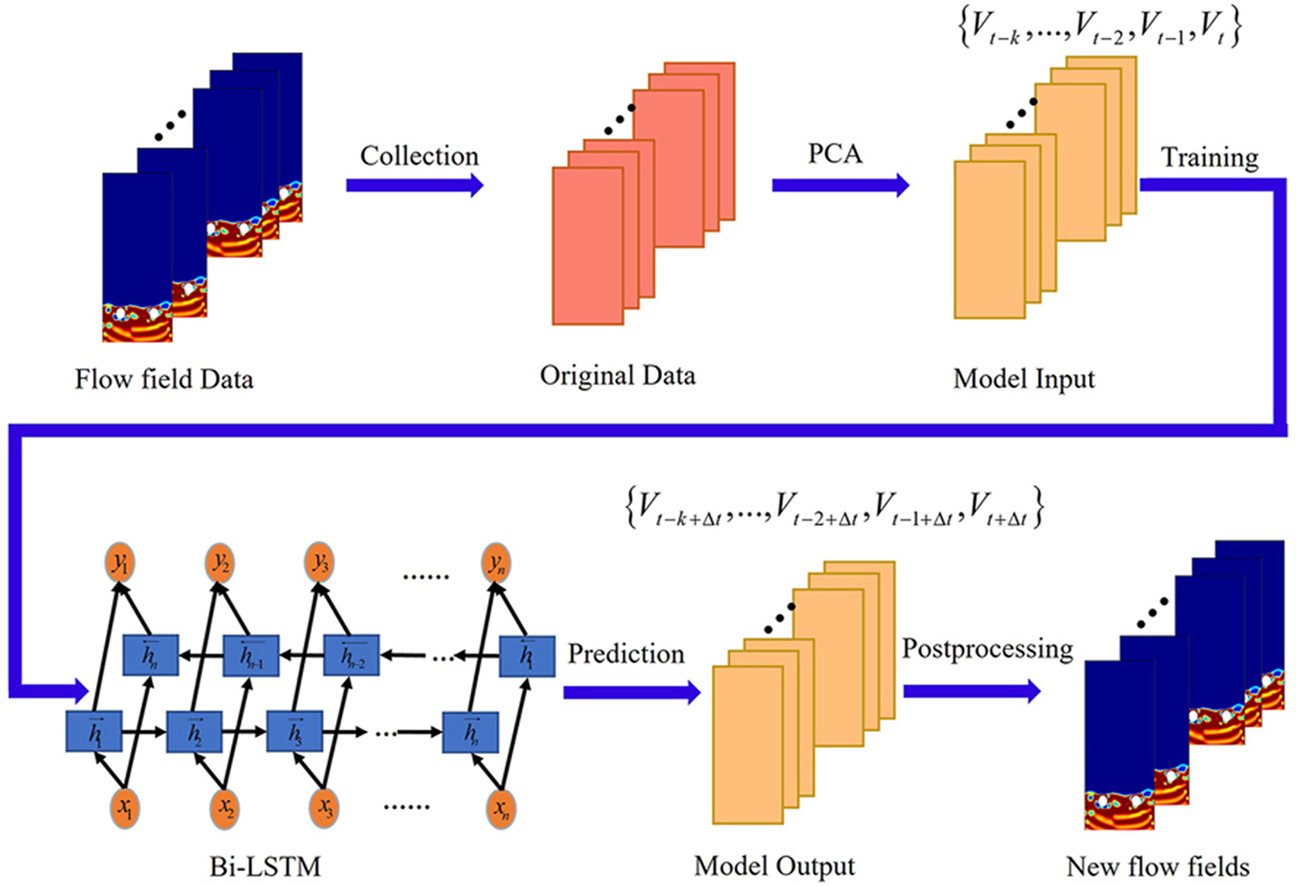