• Semi-supervised mechanism is used to improve generalization performance.
• Deep learning framework is utilized to extract features.
• An improved heuristic algorithm for optimizing the model is proposed.
• The proposed model still shows high accuracy with low sample size.
Particle entrainment is an inevitable phenomenon in pipeline systems, especially during the development and extraction phases of oil and gas wells. Accurately predicting the critical velocity for particle transport is a key focus for implementing effective sand control management. This work presents a semi-supervised learning–deep hybrid kernel extreme learning machine (SSL-DHKELM) model for predicting the critical velocity, which integrates multiple machine learning theories including the deep learning approach, which is adept at advanced feature extraction. Meanwhile, the SSL framework enhances the model's capabilities when data availability is limited. An improved slime mould algorithm is also employed to optimize the model's hyperparameters. The proposed model has high accuracy on both the sample dataset and out-of-sample data. When trained with only 10% of the data, the model's error still did not increase significantly. Additionally, this model achieves superior predictive accuracy compared to existing mechanistic models, demonstrating its impressive performance and robustness.
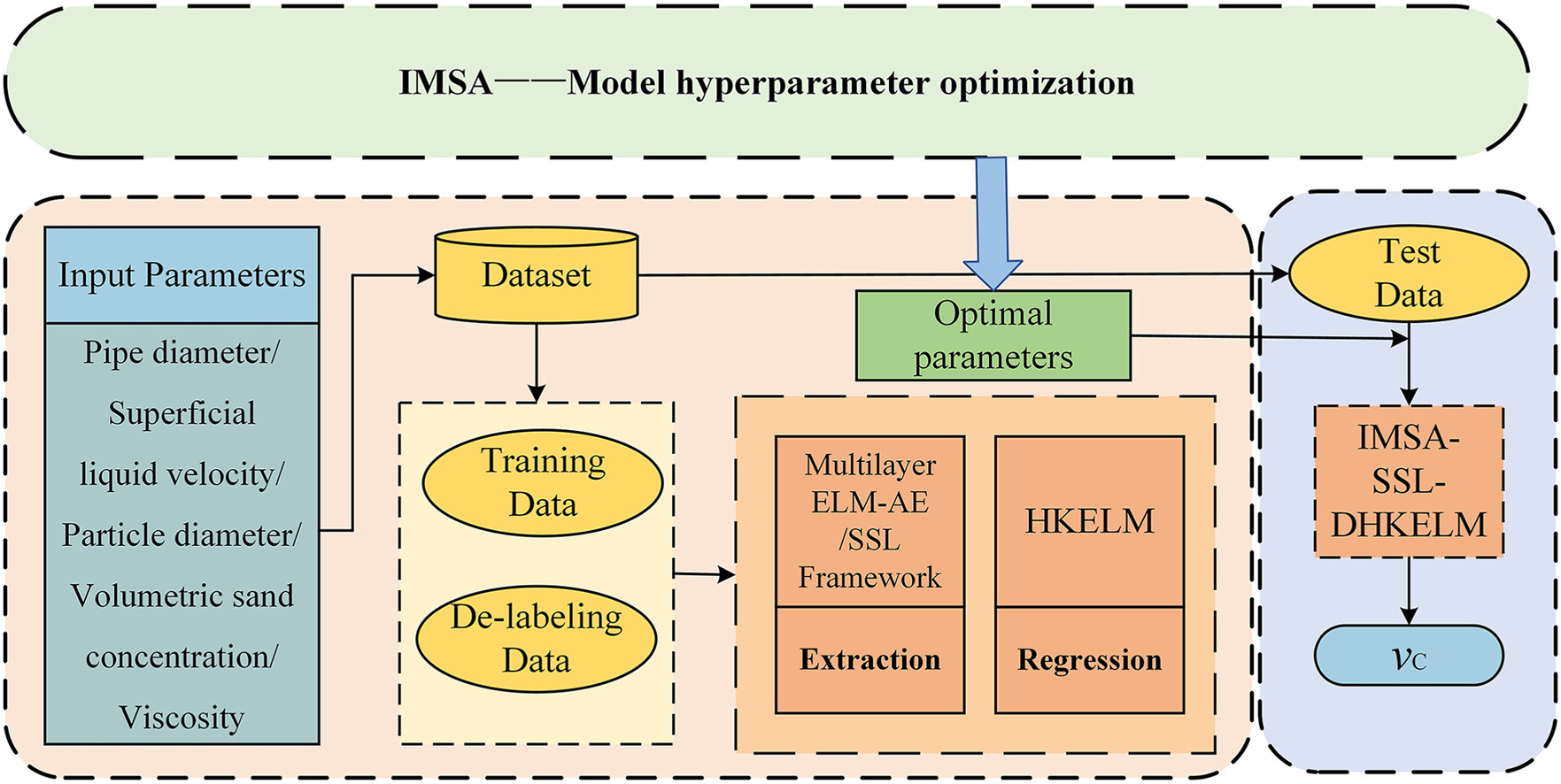