• The method incorporates Hilbert-Huang transformation and data-driven modelling.
• Experiments are conducted on a fluidized bed with different mass fractions of the mixed biomass.
• Cross correlation, EMD, and HHT are used to process the electrostatic sensing signals.
• Performances of the data-driven models (ST-BiLSTM and EMD-BiLSTM) are evaluated.
Flow dynamics of binary particles are investigated to realize the monitoring and optimization of fluidized beds. It is a challenge to accurately classify the mass fraction of mixed biomass, considering the limitations of existing techniques. The data collected from an electrostatic sensor array is analyzed. Cross correlation, empirical mode decomposition (EMD), Hilbert-Huang transform (HHT) are applied to process the signals. Under a higher mass fraction of the wood sawdust, the segregation behavior occurs, and the high energy region of HHT spectrum increases. Furthermore, two data-driven models are trained based on a hybrid wavelet scattering transform and bidirectional long short-term memory (ST-BiLSTM) network and a EMD and BiLSTM (EMD-BiLSTM) network to identify the mass fractions of the mixed biomass, with accuracies of 92% and 99%. The electrostatic sensing combined with the EMD-BiLSTM model is effective to classify the mass fraction of the mixed biomass.
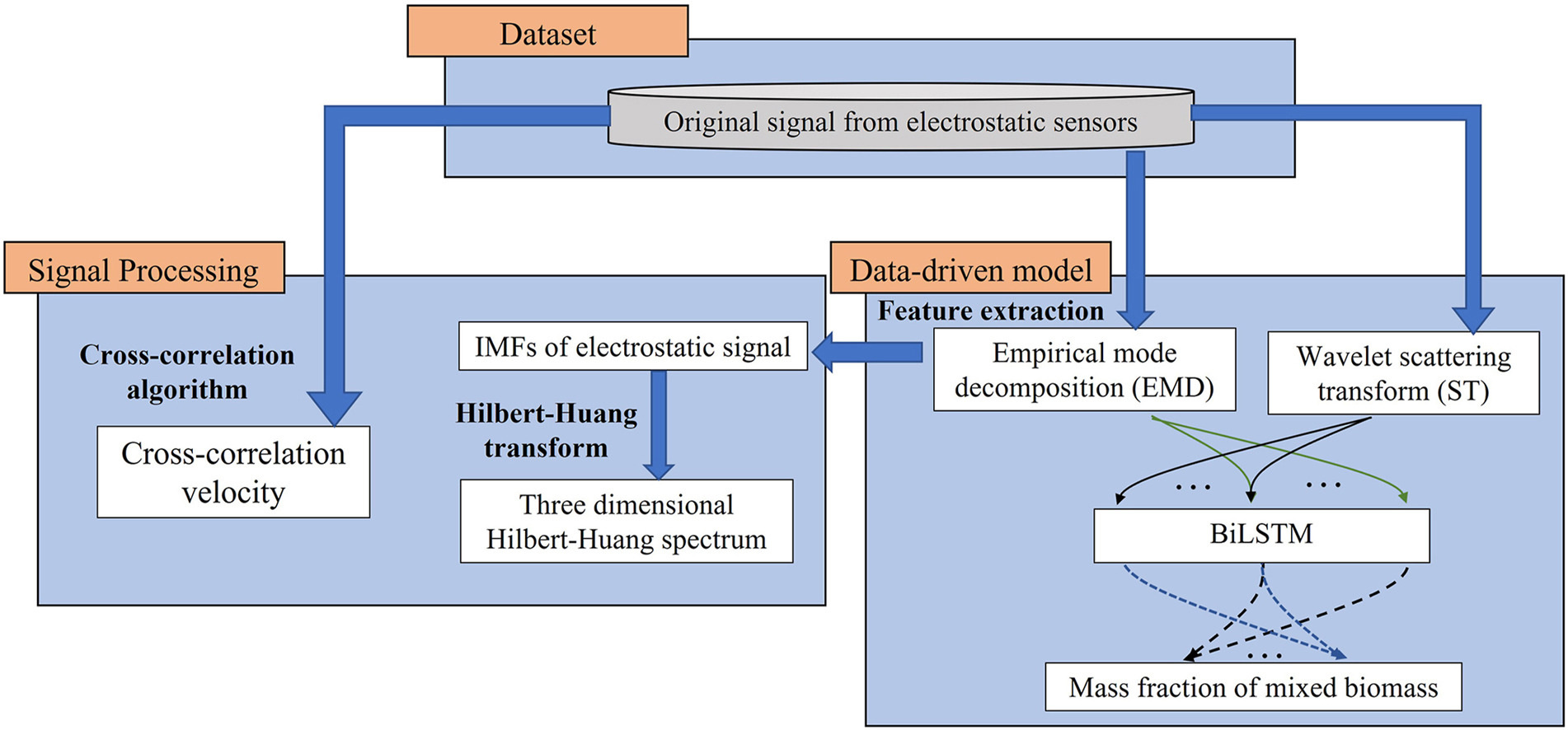