• Developed a novel method for calibration of DEM parameters of cohesive materials.
• Used Plackett-Burman method to identify vital inputs needed for DEM calibration.
• Compared performances of three calibration models: RSM, ANN, and RF.
• Verified RF model's performance consistency across various particle sizes.
• Emphasized the potential of using machine learning methods in the calibration of DEM.
This paper presents a methodology for calibrating discrete element method input parameters for simulating cohesive materials. The Plackett-Burman method was initially employed to identify the significant input parameters. Subsequently, the performances of response surface methodology (RSM), artificial neural networks (ANN), and random forest (RF) models for calibration were compared. The results demonstrated that the random forest model outperformed the two other models, achieving an RMSE of 1.89, an R-squared of 94 %, and an MAE of 1.63. The ANN model followed closely, with an RMSE of 3.12, an R-squared of 89 %, and an MAE of 2.18, while the RSM model exhibited lower performance with an RMSE of 6.84, an R-squared of 86 %, and an MAE of 5.41. This study presents a framework for enhancing the accuracy of DEM simulations. Finally, the robustness and adaptability of the calibration approach were demonstrated by applying calibrated parameters from one particle size to another.
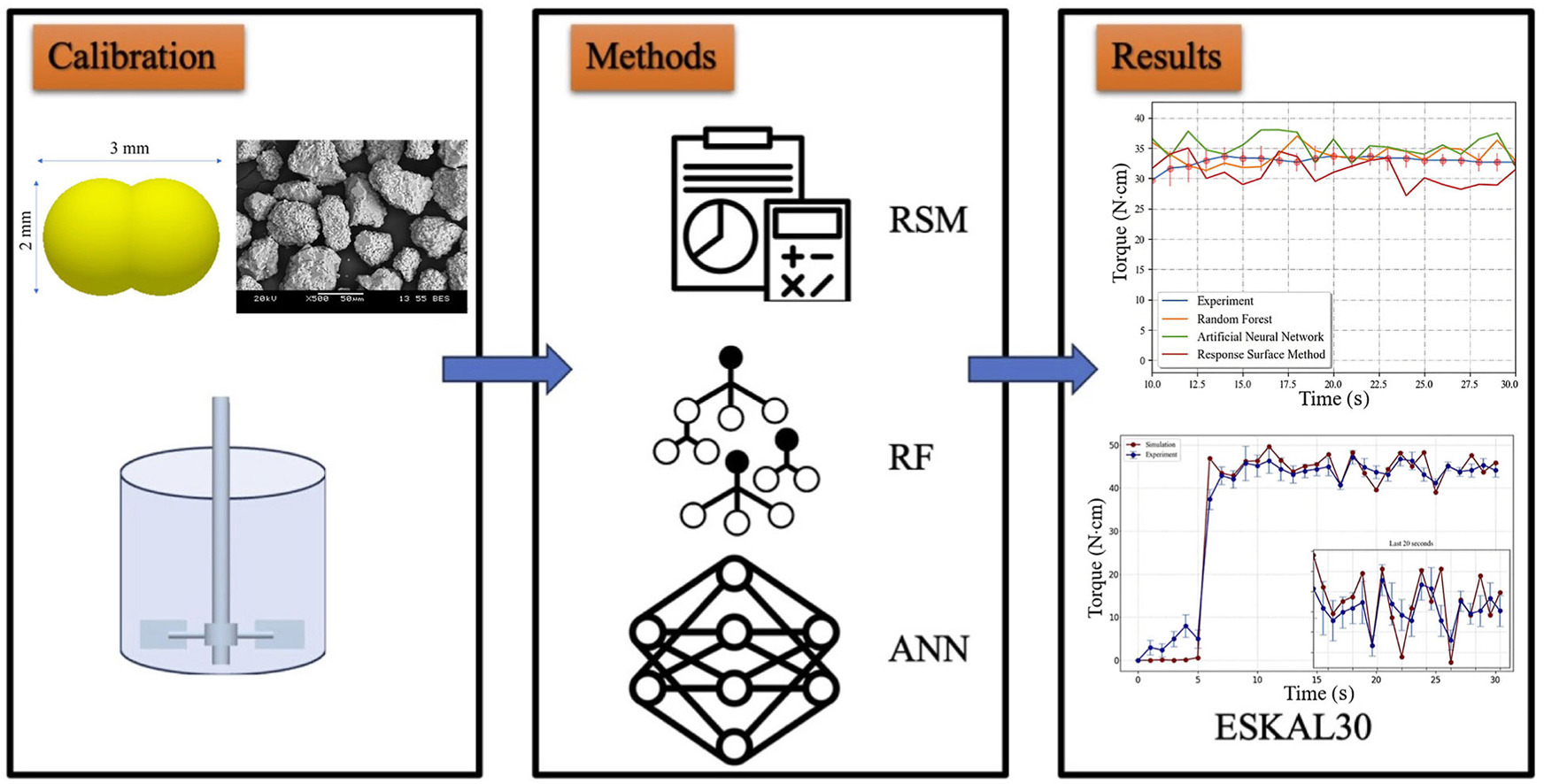