- Volumes 96-107 (2025)
-
Volumes 84-95 (2024)
-
Volume 95
Pages 1-392 (December 2024)
-
Volume 94
Pages 1-400 (November 2024)
-
Volume 93
Pages 1-376 (October 2024)
-
Volume 92
Pages 1-316 (September 2024)
-
Volume 91
Pages 1-378 (August 2024)
-
Volume 90
Pages 1-580 (July 2024)
-
Volume 89
Pages 1-278 (June 2024)
-
Volume 88
Pages 1-350 (May 2024)
-
Volume 87
Pages 1-338 (April 2024)
-
Volume 86
Pages 1-312 (March 2024)
-
Volume 85
Pages 1-334 (February 2024)
-
Volume 84
Pages 1-308 (January 2024)
-
Volume 95
-
Volumes 72-83 (2023)
-
Volume 83
Pages 1-258 (December 2023)
-
Volume 82
Pages 1-204 (November 2023)
-
Volume 81
Pages 1-188 (October 2023)
-
Volume 80
Pages 1-202 (September 2023)
-
Volume 79
Pages 1-172 (August 2023)
-
Volume 78
Pages 1-146 (July 2023)
-
Volume 77
Pages 1-152 (June 2023)
-
Volume 76
Pages 1-176 (May 2023)
-
Volume 75
Pages 1-228 (April 2023)
-
Volume 74
Pages 1-200 (March 2023)
-
Volume 73
Pages 1-138 (February 2023)
-
Volume 72
Pages 1-144 (January 2023)
-
Volume 83
-
Volumes 60-71 (2022)
-
Volume 71
Pages 1-108 (December 2022)
-
Volume 70
Pages 1-106 (November 2022)
-
Volume 69
Pages 1-122 (October 2022)
-
Volume 68
Pages 1-124 (September 2022)
-
Volume 67
Pages 1-102 (August 2022)
-
Volume 66
Pages 1-112 (July 2022)
-
Volume 65
Pages 1-138 (June 2022)
-
Volume 64
Pages 1-186 (May 2022)
-
Volume 63
Pages 1-124 (April 2022)
-
Volume 62
Pages 1-104 (March 2022)
-
Volume 61
Pages 1-120 (February 2022)
-
Volume 60
Pages 1-124 (January 2022)
-
Volume 71
- Volumes 54-59 (2021)
- Volumes 48-53 (2020)
- Volumes 42-47 (2019)
- Volumes 36-41 (2018)
- Volumes 30-35 (2017)
- Volumes 24-29 (2016)
- Volumes 18-23 (2015)
- Volumes 12-17 (2014)
- Volume 11 (2013)
- Volume 10 (2012)
- Volume 9 (2011)
- Volume 8 (2010)
- Volume 7 (2009)
- Volume 6 (2008)
- Volume 5 (2007)
- Volume 4 (2006)
- Volume 3 (2005)
- Volume 2 (2004)
- Volume 1 (2003)
• Database of 5000 points with six input features created.
• Three machine learning models were developed to predict drag coefficient.
• AdaBoost outperformed Neural Networks and Random Forest.
• Drag coefficient is highly sensitive to variations in Reynolds number and volume fraction.
Non-spherical particles are extensively encountered in the process industry such as feedstock or catalysts e.g., energy, food, pharmaceuticals, and chemicals. The design of equipment used to process these particles is highly dependent upon the accurate and reliable modeling of hydrodynamics of particulate media involved. Drag coefficient of these particles is the most significant of all parameters. A universal model to predict the drag coefficient of such particles has not yet been developed due to the diversity and complexity of particle shapes and sizes. Taking this into consideration, we propose a unique approach to model the drag coefficient of non-spherical particles using machine learning (ML) to move towards generalization. A comprehensive database of approximately five thousand data points from reliable experiments and high-resolution simulations was compiled, covering a wide range of conditions. The drag coefficient was modeled as a function of Reynolds number, sphericity, Corey Shape Factor, aspect ratio, volume fraction, and angle of incidence. Three ML techniques—Artificial Neural Networks, Random Forest, and AdaBoost—were used to train the models. All models demonstrated strong generalization when tested on unseen data. However, AdaBoost outperformed the others with the lowest MAPE (20.1%) and MRD (0.069). Additional analysis on excluded data confirmed the robust predictive abilities and generalization of the proposed model. The models were also evaluated across three flow regimes—Stokes, transitional, and turbulent—to further assess their generalization. A comparative analysis with well-known empirical correlations, such as Haider and Levenspiel and Chien, showed that all ML models outperformed traditional approaches, with AdaBoost achieving the best results. The current work demonstrates that new generated ML techniques can be reliably used to predict drag coefficient of non-spherical particles paving way towards generalization of ML approach.
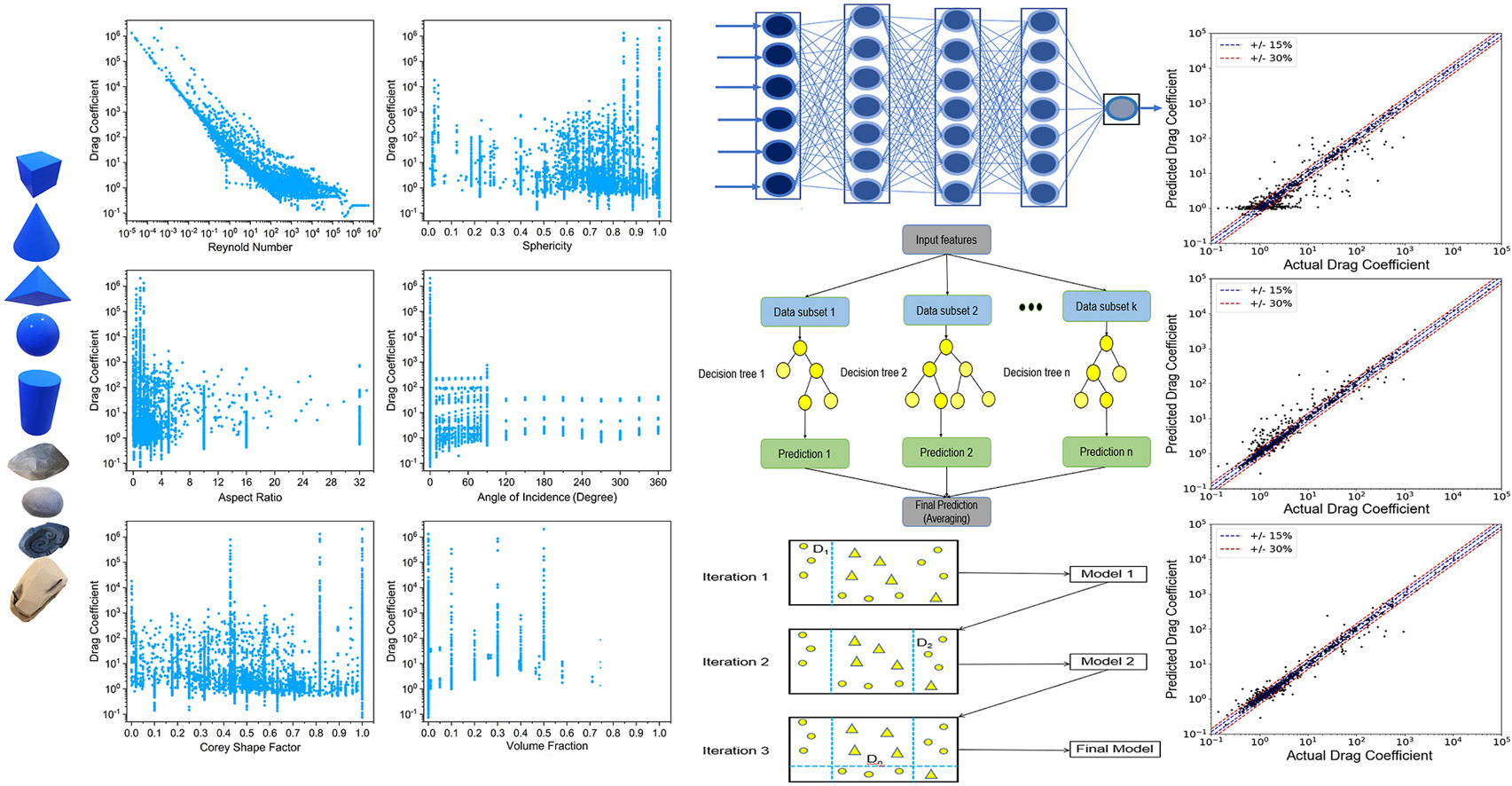