Zhipeng Wang a, Yuhang Jiang a, Yaonan Zhu b, Feng Ma c, Youzhao Wang a *, Chaoyue Zhao a, Xu Li a, Tong Zhu a *
• Machine learning and response surface methodology study of soil-agent mixing characteristics.
• Predictive performance of five machine learning models is compared.
• Improved mixing homogeneity in a new nine-axis in-situ soil remediation equipment.
• Provides reference values for the design of multi-axis soil remediation equipment.
To facilitate the recycling of polluted soils, the development of innovative multi-axial soil remediation machinery is essential for achieving a uniform blend of soil with remediation chemicals. The mean level of the steepest climb test was set using the mean level derived from the orthogonal test, and then the range of optimum values was determined based on the results of the steepest climb test, and the upper and lower bound intervals of the response surface test were set accordingly. The most optimal model is identified by applying machine learning algorithms to the response surface data. The results show that the Decision Tree model outperforms Random Forest, SVR, KNN and XG Boost in terms of accuracy and stability in predicting dual indicators. Analysis of the decision tree model yields the following optimal parameter settings: homogenisation time of 1.7 s, homogenisation spacing of 181 mm, crusher spacing of 156 mm, and speed of 113 rpm. In the final test prototype, the error rates of the machine learning prediction models were 3.01% and 3.88% respectively. The experimental data confirms that the prediction accuracy reaches a satisfactory level after applying machine learning to optimise the parameters. This study will provide a reference for the design and optimisation of new in situ multi-axial soil remediation devices.
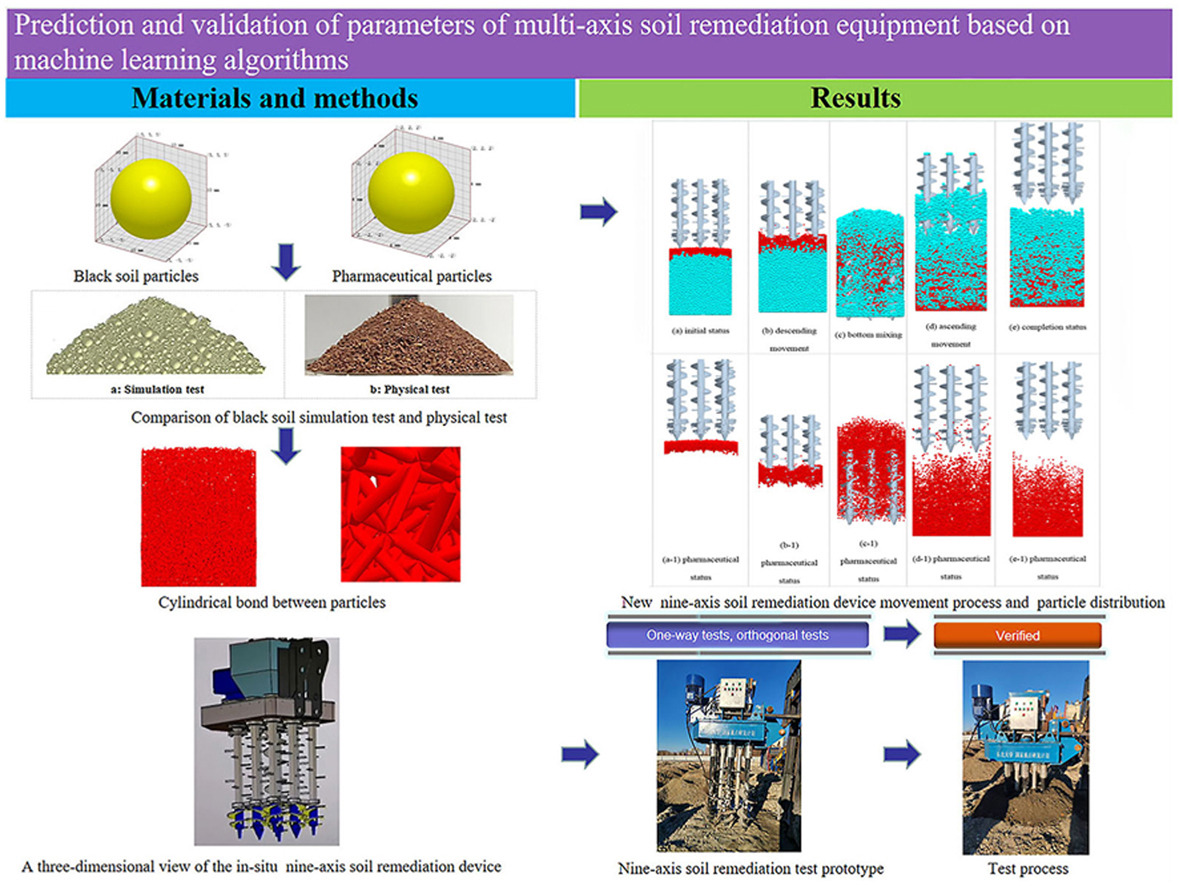